by Jennifer Hart Yim | May 12, 2015 | Big Data, Blog, Data/Analytics, Strategy
This article is part of a series of articles written by MBA students and graduates from the University of New Hampshire Peter T. Paul College of Business and Economics.
Josh Hutchins received his B.S. in Business Administration from the University of New Hampshire in 2005. He is currently pursuing his MBA at the Peter T. Paul School at the University of New Hampshire; on course to graduate in May 2016.
“Working with data is something I do every day as a financial analyst. I enjoy crunching the data and experimenting with various data analytic techniques. I’ve found that my love for playing with the data and thinking in unconventional ways has led me to be efficient and successful at work. The way data is being used is revolutionizing the way we do business. I’m glad I can be part of this wave of the future.”
The responsibility of big data
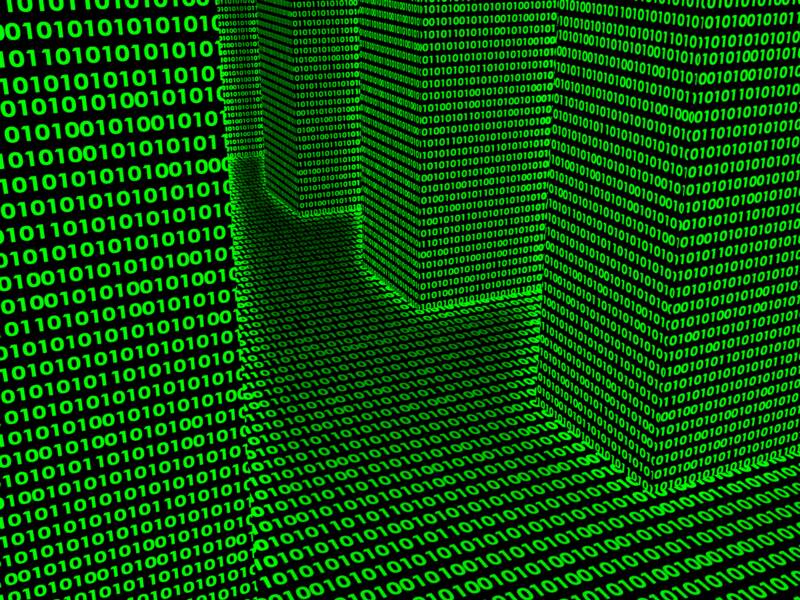
Data is coming into businesses at incredible speeds, in large quantities, and in all types of different formats. In a world full of big data it’s not just having the data – it’s what you do with it that matters. Big data analysis is becoming a very powerful tool used by companies of all sizes. Companies are analyzing and using the data in order to create sustainable business models and gain a competitive advantage over their competition. However, as one company would come to learn – with big data comes big responsibility.
Solid Gold Bomb T-Shirt Company
In 2011, Solid Gold Bomb, an Amazon Marketplace merchant based out of Australia, thought of an ingenious way to create fresh slogans for t-shirts. The main concept behind Solid Gold Bomb’s operation was that by utilizing a computer programming script, they could create clever t-shirt slogans that no one had thought of previously. The company created various t-shirt slogans that played off of the popular British WWII era phrase Keep Calm and Carry On. Under the systematic script method, Solid Gold Bombs was able to create literally millions of t-shirt offerings without the need to have them on hand in inventory. With the substantial increase in product offerings, the chances of customers stumbling upon a Solid Gold Bomb shirt increased dramatically. By utilizing this new on-demand approach to t-shirt printing, Solid Gold Bomb was able to reduce expenses, while simultaneously increasing potential revenue by offering exponential products at little additional marginal cost.
Use of ‘Big Data’
The computer script relied on the following to operate: a large pool of words, associated rule learning, and an algorithm.
Large Pool of Words – Solid Gold Bomb gathered a list of approximately 202,000 words that could be found in the dictionary. Of these words, they whittled it down to approximately 1,100 of the most popular words. Some of the words were too long to be included on a t-shirt, so the list was further culled. They settled on 700 different verbs and corresponding pronouns. These words would be used by the computer script to generate t-shirt slogans.
Associated rule learning – Associated rule learning is the degree to which two variables in a given list relate to each other. The first step in associated rule learning is to identify and isolate the most frequent variables. The second step is forming rules based on different constraints on the variables – assigning an “interestingness” factor. In the case of Solid Gold Bomb, the associated rule learning assigned an interestingness factor to verb-pronoun combinations.
Algorithm – The algorithm designed by Solid Gold Bomb was very simple. Each shirt would begin with “Keep calm and”. The algorithm script would then search through the word pool and pull back the most highly associated verb and pronoun combinations. The words would then be put into the typical format of the Keep Calm and Carry On. An image of each individual combination would be mocked up and posted to their Amazon merchant account. The process would continuously loop, creating millions of combinations.
The Big Data Blues
With one innocent mistake, Solid Gold Bomb fell apart in the blink of an eye. Amazon started getting complaints about offensive slogans on Solid Gold Bomb’s t-shirts. Images of t-shirts with phrases such as “Keep Calm and Rape Her” and “Keep Calm and Hit Her” were being sold on their Amazon merchant account. Their typical weekend orders for around 800 shirts were reduced to just 3 – few enough to count on one hand. Amazon ended up pulling their entire line of clothing, essentially putting Solid Gold Bomb out of business.
What went wrong?
While Solid Gold Bomb had a good handle on how to use data that they had gathered and how to use it to their advantage, they neglected to consider the potential hidden consequences of unintentional misuse of the data. When culling 202,000 words down to 700 useable words, words such as “rape” should have been eliminated from the useable word pool. From a high level perspective, the human mind is incapable of naming all the potential combinations of the 700 useable words without the assistance of a computer program. However, the end user needs to be aware that the computer program logic will create every potential combination based on the word pool.
Moral of the Story
Big data by itself is not beneficial to a company. The real value is in the analytics that are applied to the data. The results of the analytics can be utilized in numerous ways – to make more informed decisions, create new revenue streams, and create competitive advantages, to name a few. When a company makes the decision to utilize big data analytics, each process needs to be mapped out to have an intimate understanding of how the data will be used. In the case of Solid Gold Bomb, they failed to have this intimate understanding of how the data would be used throughout the process. As a result, they paid the ultimate price; they were not able to sustain themselves through this debacle. The morale of the story: With big data comes big responsibility. Know your data and know the potential uses of the data better. Don’t be afraid to think outside the box, but know the potential consequences.
For information about another big data faux pas, learn how Target predicted that a 16 year old girl was pregnant before her father knew.
by Elizabeth Hines | Apr 6, 2015 | Big Data, Blog, Data/Analytics
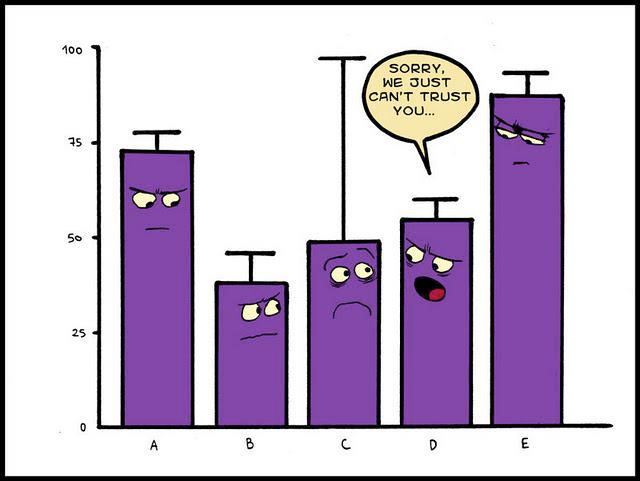
Concurrent with the extraordinary rise of the Internet of Things (IoT), predictive analytics are gaining in popularity. With an increasing number of companies learning to master the precursors to developing predictive models — namely, connecting, monitoring, and analyzing — we can safely assume the art of gleaning business intelligence from foresight will continue to grow rapidly.
Indeed, Gartner analysts put forward that “few technology areas will have greater potential to improve the financial performance and position of a commercial global enterprise than predictive analytics.” And executives seem eager to jump on the bandwagon; in a survey of executives conducted by Accenture a full 88% indicated big data analytics is a top priority for their company. Amid the promises of predictive analytics, however, we also find a number of pitfalls. Some experts caution there are situations when predictive analytics techniques can prove inadequate, if not useless.
Here, we dissect three problems most commonly encountered by companies employing predictive analytics.
Past as a poor predictor of the future
The concept of predictive analytics is predicated on the assumption that future behavior can be more or less determined by examining past behaviors; that is, predictive analytics works well in a stable environment in which the future of the business is likely to resemble its past and present. But Harvard Business School professor Clayton Christensen points out that in the event of a major disruption, the past will do a poor job of foreshadowing future events. As an example, he cites the advent of PCs and commodity servers, arguing computer vendors who specialized in minicomputers in the 1980s couldn’t possibly have predicted their sales impact, since they were innovations and there was no data to analyze.
Interpreting bias
Consider also the bias in favor of a positive result when interpreting data for predictive purposes; it is one of the most common errors in predictive analytics projects. Speaking at the 2014 Predictive Analytics World conference in Boston, John Elder, president of consulting firm Elder Research, Inc., made a good point when he noted that people “‘often look for data to justify our decisions,’ when it should be the other way around.”
Collecting and analyzing unhelpful or superfluous data
Failure to tie data efforts to operational processes can lead to an unnecessary drain of staff resources. Mining big data will do little good if the insights are not directly tied to functional procedures. More companies than we probably realize are wasting precious time and manpower on big data projects that are not adequately understood, producing trivia rather than actionable business intelligence.
To overcome these common pitfalls of predictive analytics, spend some time reviewing the sources of your data and the basic assumptions on which your predictive analytics projects are based. Because the major principle of predictive analytics is that the past behavior can forecast future behavior, keep your ear to the ground for growing industry trends or any other factors that might influence consumer behavior. Plan to revisit the source of your data frequently to determine if the sample set is representative of your future set and should continue to be used. Most importantly, regularly evaluate how your predictive analysis relates and contributes to your company’s overall goals and objectives.
What else do you think organizations can do to ward off the snags of predictive data analysis and use foresight more effectively?
by Elizabeth Hines | Apr 6, 2015 | Big Data, Blog, Data/Analytics
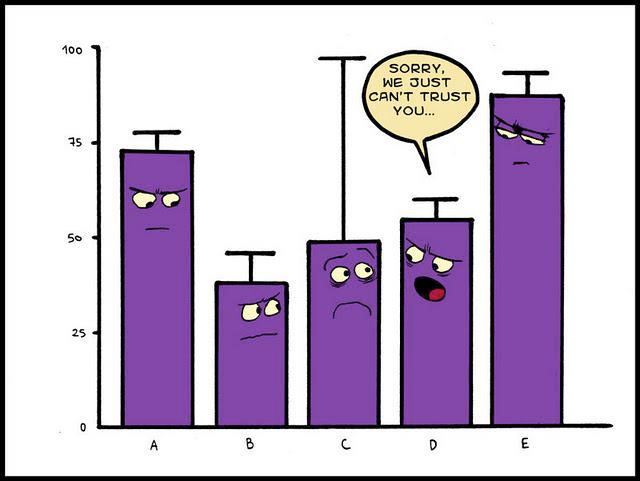
Concurrent with the extraordinary rise of the Internet of Things (IoT), predictive analytics are gaining in popularity. With an increasing number of companies learning to master the precursors to developing predictive models — namely, connecting, monitoring, and analyzing — we can safely assume the art of gleaning business intelligence from foresight will continue to grow rapidly.
Indeed, Gartner analysts put forward that “few technology areas will have greater potential to improve the financial performance and position of a commercial global enterprise than predictive analytics.” And executives seem eager to jump on the bandwagon; in a survey of executives conducted by Accenture a full 88% indicated big data analytics is a top priority for their company. Amid the promises of predictive analytics, however, we also find a number of pitfalls. Some experts caution there are situations when predictive analytics techniques can prove inadequate, if not useless.
Here, we dissect three problems most commonly encountered by companies employing predictive analytics.
Past as a poor predictor of the future
The concept of predictive analytics is predicated on the assumption that future behavior can be more or less determined by examining past behaviors; that is, predictive analytics works well in a stable environment in which the future of the business is likely to resemble its past and present. But Harvard Business School professor Clayton Christensen points out that in the event of a major disruption, the past will do a poor job of foreshadowing future events. As an example, he cites the advent of PCs and commodity servers, arguing computer vendors who specialized in minicomputers in the 1980s couldn’t possibly have predicted their sales impact, since they were innovations and there was no data to analyze.
Interpreting bias
Consider also the bias in favor of a positive result when interpreting data for predictive purposes; it is one of the most common errors in predictive analytics projects. Speaking at the 2014 Predictive Analytics World conference in Boston, John Elder, president of consulting firm Elder Research, Inc., made a good point when he noted that people “‘often look for data to justify our decisions,’ when it should be the other way around.”
Collecting and analyzing unhelpful or superfluous data
Failure to tie data efforts to operational processes can lead to an unnecessary drain of staff resources. Mining big data will do little good if the insights are not directly tied to functional procedures. More companies than we probably realize are wasting precious time and manpower on big data projects that are not adequately understood, producing trivia rather than actionable business intelligence.
To overcome these common pitfalls of predictive analytics, spend some time reviewing the sources of your data and the basic assumptions on which your predictive analytics projects are based. Because the major principle of predictive analytics is that the past behavior can forecast future behavior, keep your ear to the ground for growing industry trends or any other factors that might influence consumer behavior. Plan to revisit the source of your data frequently to determine if the sample set is representative of your future set and should continue to be used. Most importantly, regularly evaluate how your predictive analysis relates and contributes to your company’s overall goals and objectives.
What else do you think organizations can do to ward off the snags of predictive data analysis and use foresight more effectively?
by Elizabeth Hines | Apr 1, 2015 | Big Data, Blog, Data/Analytics, Logistics, Supply Chain
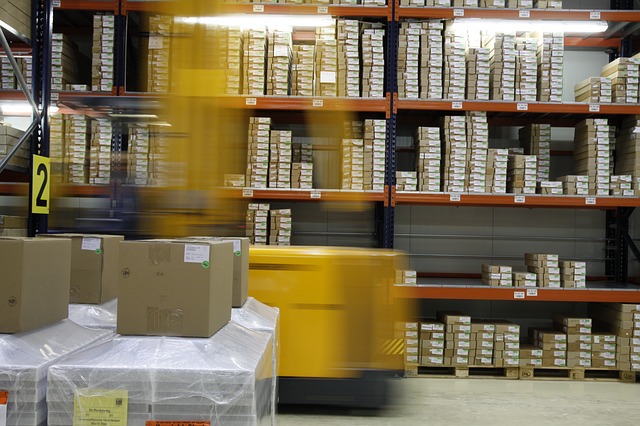
A distribution center struggling with a high number of forklift truck impacts found a way to leverage big data to address a nagging, costly warehouse issue. The company had installed a telematics solution on its forklift trucks, but could not determine the cause of the impacts. The time and location of impacts were known, as well as the identity of the drivers involved, but the company still needed to pull in more data sources for an effective assessment.
Forget for a moment the potential of adopting big data analytics throughout the entire supply chain and consider instead how big data can untangle and integrate seemingly unrelated masses of data to solve small problems in a warehouse or distribution center. That’s exactly what this company did.
By analyzing the link between environmental factors inside the distribution center and the forklift impact records, the culprit was swiftly identified: fast-moving thunderstorms that caused the humidity level to rise so quickly that the dehumidifiers could not keep up, increasing the risk of drivers losing control on the slippery concrete floor. That knowledge helped the company prevent sliding accidents by using a function of the telematics solution to reduce the maximum speed of the trucks when the humidity hit a certain level.
Indeed, distribution centers and warehouses present ideal environments — microcosms — for big data applications. Modern facilities are loaded with sensors and detectors to track every pallet and every piece of material handling equipment in real-time. Managers see the benefits in increased productivity, improved inventory flow, optimized equipment usage, and more. However, for that Eureka moment, organizations should also apply big data analytics across these multiple sources of data to uncover patterns that will drive even more, and perhaps surprising, operational improvements.
Rather than looking at data in isolation, a holistic approach holds significantly more power. Managers typically keep careful track of the performance of lift trucks, batteries, and chargers. But it is not until those entities are reviewed as a single system and matched with data coming off the lift trucks that a new level of revelations can be had.
Look for big data analytics to further raise the IQ of our “smart” warehouses and DCs. Inbound Logistics sums it up this way: “Accessing the right information to make smart decisions in the warehouse is one main reason why the demand for big data has grown so much — and so rapidly — in the distribution sector.”
Do you think distribution center and warehouse managers do enough to leverage big data?
by Elizabeth Hines | Apr 1, 2015 | Big Data, Blog, Data/Analytics, Logistics, Supply Chain
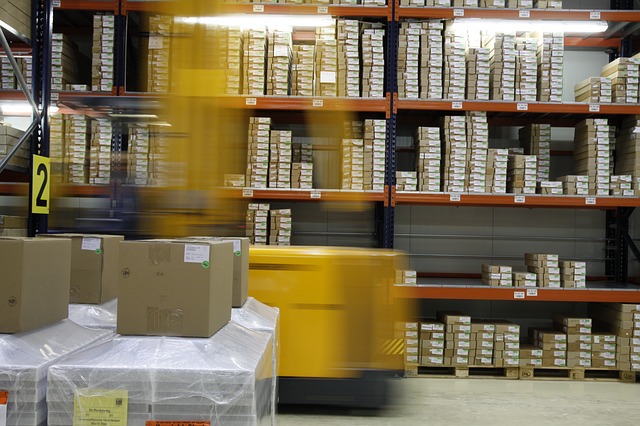
A distribution center struggling with a high number of forklift truck impacts found a way to leverage big data to address a nagging, costly warehouse issue. The company had installed a telematics solution on its forklift trucks, but could not determine the cause of the impacts. The time and location of impacts were known, as well as the identity of the drivers involved, but the company still needed to pull in more data sources for an effective assessment.
Forget for a moment the potential of adopting big data analytics throughout the entire supply chain and consider instead how big data can untangle and integrate seemingly unrelated masses of data to solve small problems in a warehouse or distribution center. That’s exactly what this company did.
By analyzing the link between environmental factors inside the distribution center and the forklift impact records, the culprit was swiftly identified: fast-moving thunderstorms that caused the humidity level to rise so quickly that the dehumidifiers could not keep up, increasing the risk of drivers losing control on the slippery concrete floor. That knowledge helped the company prevent sliding accidents by using a function of the telematics solution to reduce the maximum speed of the trucks when the humidity hit a certain level.
Indeed, distribution centers and warehouses present ideal environments — microcosms — for big data applications. Modern facilities are loaded with sensors and detectors to track every pallet and every piece of material handling equipment in real-time. Managers see the benefits in increased productivity, improved inventory flow, optimized equipment usage, and more. However, for that Eureka moment, organizations should also apply big data analytics across these multiple sources of data to uncover patterns that will drive even more, and perhaps surprising, operational improvements.
Rather than looking at data in isolation, a holistic approach holds significantly more power. Managers typically keep careful track of the performance of lift trucks, batteries, and chargers. But it is not until those entities are reviewed as a single system and matched with data coming off the lift trucks that a new level of revelations can be had.
Look for big data analytics to further raise the IQ of our “smart” warehouses and DCs. Inbound Logistics sums it up this way: “Accessing the right information to make smart decisions in the warehouse is one main reason why the demand for big data has grown so much — and so rapidly — in the distribution sector.”
Do you think distribution center and warehouse managers do enough to leverage big data?