by Fronetics | Jul 9, 2015 | Blog, Data/Analytics, Marketing, Social Media
In what seemed like a curious social experiment, KLM Royal Dutch Airline harnessed the popularity of social media and launched their “Meet and Seat” program in 2012. The service gives passengers the option to link their social media profiles to their boarding information during the ticket purchase process. Once a traveler’s profile is linked, they gain access to other social profiles of travelers that have also opted in to the service. Underling the concept is the notion that travelers will choose seatmates based on the profiles of users with similar business or leisure interests. While KLM reports that over 50,000 flyers have utilized the service since its inception – enough for KLM to deem the program successful – what’s discernable from KLM’s efforts is that social media has become a major player in today’s business world.
With a reported 8 out of 10 businesses using social media in the communication between their brand and their audiences, companies are spending more marketing dollars on social efforts with increasing regularity. But with its fairly recent introduction to the business world, many companies are struggling to determine whether their efforts, and their investments, are paying off. Fortunately, by focusing on a few key metrics, your company can start to understand which of your social efforts are adding value and, just as important, which ones are not.
Here are the 4 social metrics that matter:
Source Reports
The most common remark about measurement you’ll hear social media marketing experts say is that social media efforts shouldn’t be solely measured in likes and follows. It’s hard to connect the number of likes and follows to financial business metrics, so thinking about the success of your channels in different terms gives a more insightful report about how your social channels are performing. HubSpot recommends tracking the network source of your web traffic, leads, and customers to determine how your social platforms are performing.
Track: # of visits, leads, and customers for each of your social channels
Engagement and Amplification
“No man is an island,” wrote John Donne in his poem. His summation about the nature of humans is quite evident in social media. Indeed, one of the greatest benefits of social media is that it is by its very nature, social. For companies, social media exponentially expands the reach of its content by playing on the communal nature of these networks. If a company has 2,000 followers on Twitter, and each of them shared that company’s content with 200 of their followers, the amplification of its content has expanded to 200,000 users. Your business can leverage these immense networks by maintaining consistency in the quality and value of your content as this strategy will naturally inspire followers to share and repost your content. Track the types of content that drives the most amplification and identify trends in popular publishing times, then refine your efforts based on your analysis. Remember, as a business using social media, your end goal is action, not eyes. In other words, what’s important to your efforts is engagement. Pay close attention to how your followers are interacting with your content.
Track: # of Facebook Post Shares, # of Retweets, # of Twitter Mentions
Lead Generation
Put simply, lead generation activity lets you know whether your social content is generating leads and creating opportunities for your business. At the end of the day, your social efforts don’t matter if they aren’t playing a part in driving new business. Of particular value to your business is your conversion rate. Knowing how often content consumption results in a new lead will help you to build a more successful overall content strategy. Is your new infographic converting visitors to leads at a higher rate than your verbose Facebook posts? That might be a sign that your audience responds better to image-heavy content.
Track: # of form submissions, # of email subscribers, conversion rate
Return on Investment
If one of your company’s objectives for its social media participation is to drive growth, your company’s ROI calculation is one of the most important measurements of your social efforts. This measurement determines what impact your social content is having on customer acquisition and sales. Just as you would for all other marketing efforts, you need to know whether or not the investment you’re making in social media is producing a positive return for your business. To find your investment, start by calculating the total of your investment, your overhead factor, and your miscellaneous costs. To calculate your return, you’ll need to know your leads per month, your lead conversion rate, your average lifetime customer value, and average profit margin. Let’s take a look at how those factor into your ROI calculation in the example below.
To Calculate Investment
- Multiply the hours per month needed to create the content by the hourly rate of the employees used to create the content.
- Multiply the result by the overhead factor, taking into account rent, insurance, utilities, etc. (typically 50%)
- Add all other costs, such as design fees, hosting fees, subscriptions, software, etc. You may choose to allocate them to a specific piece of content or amortize them monthly and spread the costs evenly across each piece of content.
Calculation: At 40 hours/month at $40/hour to produce a corporate blog, multiplied by a 50% overhead factor. Add in $1k/month for design, $100/month for hosting, and $100/month for miscellaneous fees.
Total Investment in Monthly Blog = $3,600
To Calculate Return
Multiply your leads per month by your lead conversion rate, average lifetime customer value, and average profit margin.
Calculation: You collect 25 leads a month from your blog. At a 20% lead conversion rate, you’ll generate five new customers. Assume a $3,000 average lifetime customer value and a 30% average profit margin.
Total Monthly Blogging Return = $4,500
To Calculate Return on Investment
Subtract the investment from the return. Then, divide by the investment.
Calculation:
$4,500 – $3,600 = 900
900/3,600 = .25
ROI = 25%
Nearly 70% of communications professionals report dissatisfaction in how they’re measuring their social efforts. That’s understandable, social can be cumbersome to analyze. But looking at activity through the lens of your business objectives should really get to the heart of why your company participates in social media. In your work to distinguish your company from the thousands of others in the sea of social, seek to create rich and robust social experiences for your followers by connecting them with relevant and valuable content. Then measure that user activity and engagement to see how it is helping grow your business.
Fronetics Strategic Advisors is a leading management consulting firm. Our firm works with companies to identify and execute strategies for growth and value creation.
Whether it is a wholesale food distributor seeking guidance on how to define and execute corporate strategy; a telematics firm needing high quality content on a consistent basis; a real estate firm looking for a marketing partner; or a supply chain firm in need of interim management, our clients rely on Fronetics to help them navigate through critical junctures, meet their toughest challenges, and take advantage of opportunities. We deliver high-impact results.
We advise and work with companies on their most critical issues and opportunities: strategy, marketing, organization, talent acquisition, performance management, and M&A support.
We have deep expertise and a proven track record in a broad range of industries including: supply chain, real estate, software, and logistics.
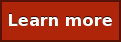
by Fronetics | Jul 6, 2015 | Big Data, Blog, Data/Analytics, Internet of Things, Logistics, Marketing, Strategy, Supply Chain
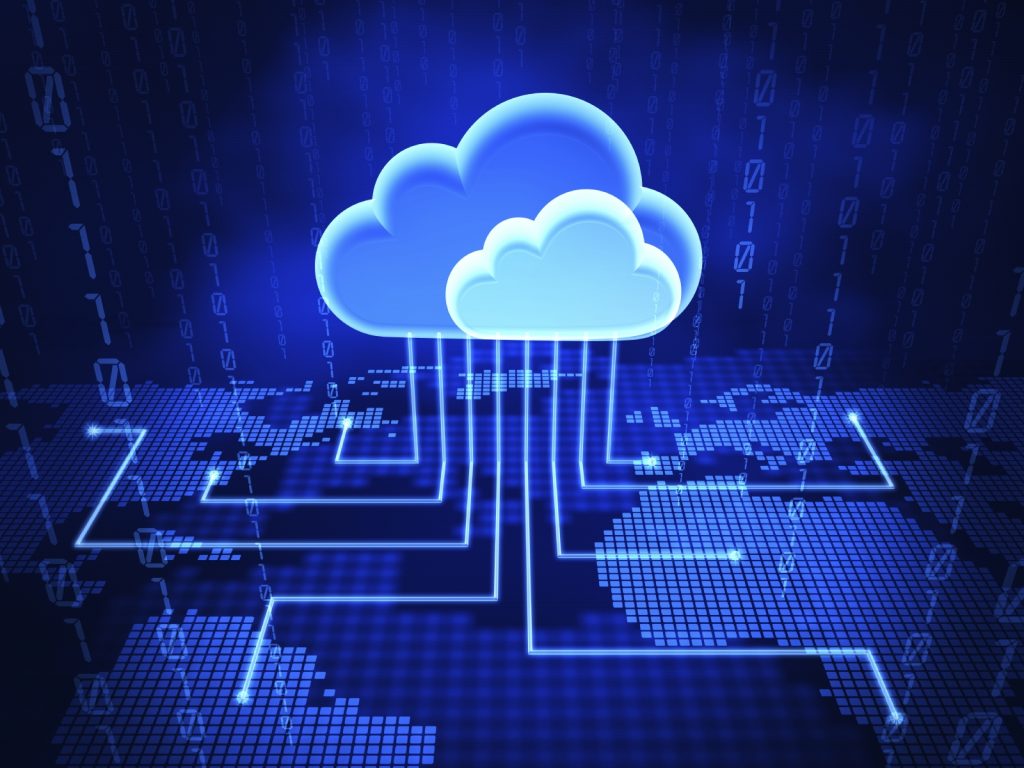
The battle for competitiveness in the cloud.
In this age of radical transformation for supply chains, top companies are tying together prevailing concepts, like big data and the Internet of Things (IoT), with cloud-based computing. Supply chains are being reimagined as digital networks that track not only physical goods, but also people, data, and money. As such, global technology giants continue to invest heavily in cloud computing.
The Chinese e-commerce company Alibaba Group Holding, Ltd. recently announced that it is launching its first international venture – a cloud computing hub in Silicon Valley, proving the fierce competition for market share is stiffening both globally and on the home turf. Notably, all five top-ranked companies listed by the 2013 Strategy & Global Information, Communications and Technology 50 study bet their future on cloud technologies. The companies (IBM, Microsoft, SAP, Oracle, and Cisco Systems) could not, until recently, be perceived as direct competitors, and now they all wield cloud-based portfolios as their competitive weapon of choice.
Analysts note:
The industry leaders are seeking dominant positions, wanting to become the kings of the cloud. As a group, they are putting distance between themselves and the second tier of followers.
For further proof data analytics is driving competition for cloud customers, we can look to a new report by Market & Research that shows data analytics and cloud computing are expected to record a combined growth of 26% annually over the next five years. The implication is that demand is hardly going to lessen as an increasing number of organizations need cloud solutions to manage and store the huge amounts of data that they use to transform manufacturing processes, fine-tune supply chains, forecast customer behavior, and optimize inventories – to name a fraction of potential applications.
And cloud-based computing is even moving the needle of interest in industries that seem inherently averse to making data and information more easily accessible. For example, concerns about data encryption, auditing controls, and transparency have stymied the adoption of cloud-based computing in the financial industry. According to a report by the Cloud Security Alliance, only 28% of American financial institutions have a cloud-based strategy in place, but as a sponsor of the report, Dr. Chenxi Wang, vice president of Cloud Security and Strategy at CipherCloud points out:
Cloud has made solid inroads in this industry with many firms looking to harnessing the power of cloud. There’s plenty of room for growth, particularly for providers who can fill the void for the auditing and data protection controls that are at the top of respondents’ cloud wish list.
Meanwhile, the public cloud services market alone could grow into a $100 billion industry by 2017, according to researcher IDC. Is your business prepared to leverage cloud computing for its supply chain activities? It’s coming, ready or not.
Fronetics Strategic Advisors is a leading management consulting firm. Our firm works with companies to identify and execute strategies for growth and value creation.
Whether it is a wholesale food distributor seeking guidance on how to define and execute corporate strategy; a telematics firm needing high quality content on a consistent basis; a real estate firm looking for a marketing partner; or a supply chain firm in need of interim management, our clients rely on Fronetics to help them navigate through critical junctures, meet their toughest challenges, and take advantage of opportunities. We deliver high-impact results.
We advise and work with companies on their most critical issues and opportunities: strategy, marketing, organization, talent acquisition, performance management, and M&A support.
We have deep expertise and a proven track record in a broad range of industries including: supply chain, real estate, software, and logistics.
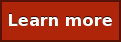
by Fronetics | Jun 25, 2015 | Big Data, Blog, Data/Analytics, Internet of Things, Marketing
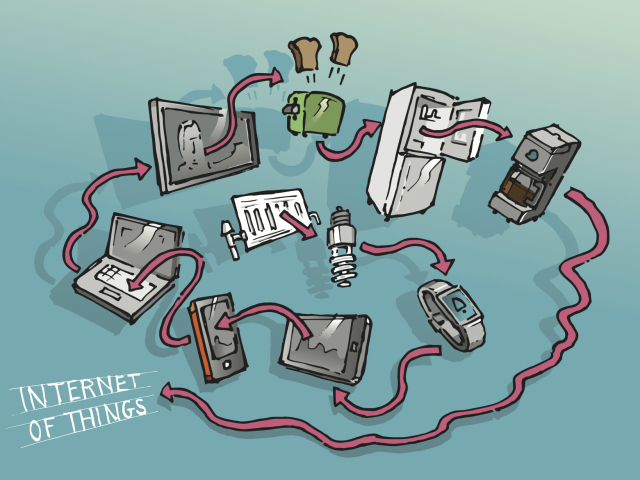
From coffee makers to urban design, the Internet of Things (IoT) is affecting change in virtually all aspects of daily life. And even though the IoT is still at the early-adopter stage, in just five years 50 billion devices are projected to be connected to the Internet, generating an estimated $2 trillion to $14 trillion in value. Expectations are running high, so high, in fact, that Gartner ranked IoT at the top of its 2014 Hype Cycle for Emerging Technologies.
Companies are naturally eager to get a piece of the action with as many as three out of four exploring how IoT technologies could fit into their business operations. Too many times, however, companies end up with mountains of data and no actionable information. Entry into the IoT should come with warnings: analyze the data or prepare to be disappointed. Or, data without analytics is nothing but noise.
But unless businesses turn the focus away from pure data collection to data analysis, investments in IoT technologies are doomed to produce disappointing results. To be truly useful, the data should do more than “look pretty on a dashboard,” as Steven Sarracino, founder of Activant Capital Group, LLC, in Greenwich, Conn., pointed out.
Vendors of sensor technologies would simultaneously be wise to take their services beyond singing the virtues of amassing data to showing their clients how to make sensor-driven decisions. In fact, the need for more guidance was underscored in a fleet report by Tracking Automotive Technology: TU Automotive (previously Telematics Update). Vendors should, according to the report, present the data in a digestible format to assist overwhelmed end-users. While purely monitoring the performance of a forklift, for example, provides value, it is not until the data is analyzed and acted upon that maximum ROI is achieved. In the case of a forklift fleet, it might entail optimizing routes in the warehouse or performing preventive maintenance. As another example, the retail sector can apply analytics to data collected by security cameras and Wi-Fi beacons to help retailers understand what types of displays catch customers’ attention.
The adoption of IoT technologies will likely come easier to industries such as manufacturing and supply chain which already connect machinery and fleets with Internet-enabled sensors or devices. Smart grid technologies also hold a lot of promise for public utilities based on current industry trends, connecting countless data points for continuous monitoring and proactive management of the power supply. However, until companies are able to adequately apply analytics to squeeze value out of their investments, it may be a while before IoT technologies reach critical mass.
by Fronetics | Jun 15, 2015 | Blog, Content Marketing, Data/Analytics, Marketing
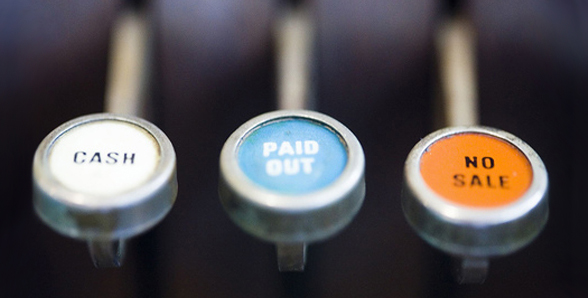
It’s impossible not to recognize that the business world is changing. Whether it’s the fall of the travel agent as people migrate towards online booking, or the irrelevance of the compact disc as Spotify and iTunes changed the music industry, or how advertising is done. Outbound marketing, such as print ads, TV ads, banner ads, trade shows, telemarketing, and direct mail are no longer what consumers are requiring. According to Forbes, many brands are moving their advertising budgets from television to online videos. The Content Marketing Institute reports that 8 out of 10 people identify themselves as blog readers, and 23% of all time spent online is spent on social media sites. With the rise of the blog, companies have gotten smart about how to reach their current and potential consumers.
In the B2B world things are changing, too, with many executives wanting to gain information through other mediums. The Content Marketing Institute also reports that a majority (80%) of business decision-makers prefer to get information from articles rather than through advertisements.
We know that inbound marketing is effective in garnering consumers’ attention. It’s aligned with a generation of people who want to be educated about the products they’re buying and who are willing to search for those products online. Even with all of this known, it’s important to ask: what is the ROI when it comes to content marketing?
According to Search Engine Journal, inbound leads cost 60% less than outbound leads. In the Harvard Business Review article, How to Profit from “Lean Advertising”, the shoe company DC Shoes is profiled as a model for inbound marketing. In an industry where star athletes are profiled in big-production advertisements via TV commercials, billboards, and magazine ads, the skateboard shoe company decided to take a different route. According to the 2013 HBS article, “Over the past four years they have gotten more than 180 million views—and in 2011 alone, sales jumped 15%. One was YouTube’s most-shared video of 2011; another garnered a million views in its first 24 hours. Paying online media for this type of exposure would cost upward of $5 million.”
Like any new tack in business decisions, relying on case studies from other businesses is helpful, but cost needs to be considered. In order to calculate ROI the cost of content marketing needs to be assessed:
- salaries (if going in-house)
- marketing agency or contractor services
- additional overhead
- distribution costs
- design and publication software
After those costs have been calculated, the next step is to subtract that number from the revenue generated. The Guardian has put forth its simple content marketing ROI calculator:
(Revenue Generated – Cost of Content Marketing) / Cost of Content Marketing = ROI
According to the newspaper, “A simple calculation could say that you drove 1000 visits through a piece of content, and Google Ads would have cost £1 per click, e.g. £1000 to equal the same. If the content only cost £500, you have a saving!”
But with most seemingly simple things, there’s complexity underneath. Dig deeper and ask more questions. Is the money you’re spending on inbound marketing deterring other, less obvious, costs? Would it have cost you more through outbound marketing methods to achieve that same level of visibility than through inbound marketing solutions? Is inbound marketing bringing in customers or closing a deal more quickly than alternative methods (time is money, after all)? Is inbound marketing cutting down the need for staffing in other areas, such as support staff to manage inquiries or support calls?
Some incalculable values from inbound marketing, like consumer preferences, content intelligence, customer relationship strategies, and branding can be hard to tie to a number, but over time you will see that your ROI will become more clear to you as you generate leads, turn leads into customers, and see the result in the form of money gained (American dollars or British pounds!).
by Jennifer Hart Yim | May 12, 2015 | Big Data, Blog, Data/Analytics, Strategy
This article is part of a series of articles written by MBA students and graduates from the University of New Hampshire Peter T. Paul College of Business and Economics.
Josh Hutchins received his B.S. in Business Administration from the University of New Hampshire in 2005. He is currently pursuing his MBA at the Peter T. Paul School at the University of New Hampshire; on course to graduate in May 2016.
“Working with data is something I do every day as a financial analyst. I enjoy crunching the data and experimenting with various data analytic techniques. I’ve found that my love for playing with the data and thinking in unconventional ways has led me to be efficient and successful at work. The way data is being used is revolutionizing the way we do business. I’m glad I can be part of this wave of the future.”
The responsibility of big data
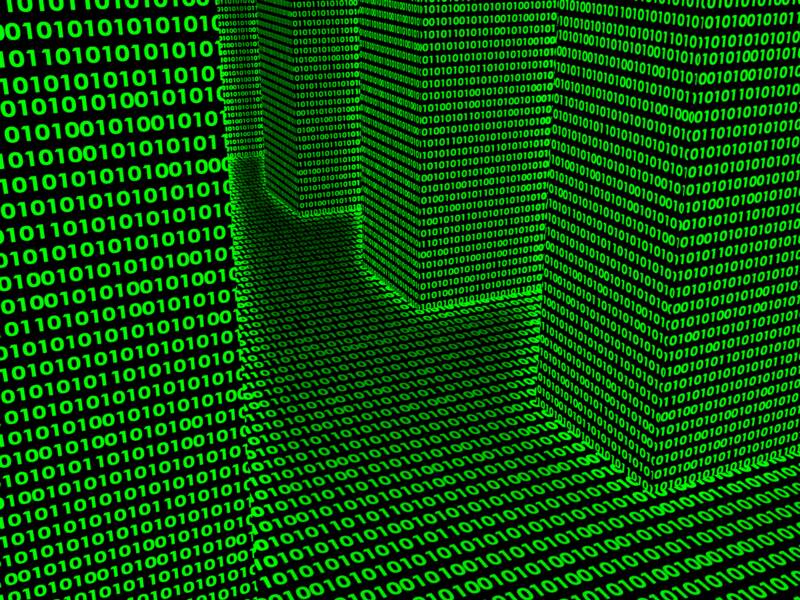
Data is coming into businesses at incredible speeds, in large quantities, and in all types of different formats. In a world full of big data it’s not just having the data – it’s what you do with it that matters. Big data analysis is becoming a very powerful tool used by companies of all sizes. Companies are analyzing and using the data in order to create sustainable business models and gain a competitive advantage over their competition. However, as one company would come to learn – with big data comes big responsibility.
Solid Gold Bomb T-Shirt Company
In 2011, Solid Gold Bomb, an Amazon Marketplace merchant based out of Australia, thought of an ingenious way to create fresh slogans for t-shirts. The main concept behind Solid Gold Bomb’s operation was that by utilizing a computer programming script, they could create clever t-shirt slogans that no one had thought of previously. The company created various t-shirt slogans that played off of the popular British WWII era phrase Keep Calm and Carry On. Under the systematic script method, Solid Gold Bombs was able to create literally millions of t-shirt offerings without the need to have them on hand in inventory. With the substantial increase in product offerings, the chances of customers stumbling upon a Solid Gold Bomb shirt increased dramatically. By utilizing this new on-demand approach to t-shirt printing, Solid Gold Bomb was able to reduce expenses, while simultaneously increasing potential revenue by offering exponential products at little additional marginal cost.
Use of ‘Big Data’
The computer script relied on the following to operate: a large pool of words, associated rule learning, and an algorithm.
Large Pool of Words – Solid Gold Bomb gathered a list of approximately 202,000 words that could be found in the dictionary. Of these words, they whittled it down to approximately 1,100 of the most popular words. Some of the words were too long to be included on a t-shirt, so the list was further culled. They settled on 700 different verbs and corresponding pronouns. These words would be used by the computer script to generate t-shirt slogans.
Associated rule learning – Associated rule learning is the degree to which two variables in a given list relate to each other. The first step in associated rule learning is to identify and isolate the most frequent variables. The second step is forming rules based on different constraints on the variables – assigning an “interestingness” factor. In the case of Solid Gold Bomb, the associated rule learning assigned an interestingness factor to verb-pronoun combinations.
Algorithm – The algorithm designed by Solid Gold Bomb was very simple. Each shirt would begin with “Keep calm and”. The algorithm script would then search through the word pool and pull back the most highly associated verb and pronoun combinations. The words would then be put into the typical format of the Keep Calm and Carry On. An image of each individual combination would be mocked up and posted to their Amazon merchant account. The process would continuously loop, creating millions of combinations.
The Big Data Blues
With one innocent mistake, Solid Gold Bomb fell apart in the blink of an eye. Amazon started getting complaints about offensive slogans on Solid Gold Bomb’s t-shirts. Images of t-shirts with phrases such as “Keep Calm and Rape Her” and “Keep Calm and Hit Her” were being sold on their Amazon merchant account. Their typical weekend orders for around 800 shirts were reduced to just 3 – few enough to count on one hand. Amazon ended up pulling their entire line of clothing, essentially putting Solid Gold Bomb out of business.
What went wrong?
While Solid Gold Bomb had a good handle on how to use data that they had gathered and how to use it to their advantage, they neglected to consider the potential hidden consequences of unintentional misuse of the data. When culling 202,000 words down to 700 useable words, words such as “rape” should have been eliminated from the useable word pool. From a high level perspective, the human mind is incapable of naming all the potential combinations of the 700 useable words without the assistance of a computer program. However, the end user needs to be aware that the computer program logic will create every potential combination based on the word pool.
Moral of the Story
Big data by itself is not beneficial to a company. The real value is in the analytics that are applied to the data. The results of the analytics can be utilized in numerous ways – to make more informed decisions, create new revenue streams, and create competitive advantages, to name a few. When a company makes the decision to utilize big data analytics, each process needs to be mapped out to have an intimate understanding of how the data will be used. In the case of Solid Gold Bomb, they failed to have this intimate understanding of how the data would be used throughout the process. As a result, they paid the ultimate price; they were not able to sustain themselves through this debacle. The morale of the story: With big data comes big responsibility. Know your data and know the potential uses of the data better. Don’t be afraid to think outside the box, but know the potential consequences.
For information about another big data faux pas, learn how Target predicted that a 16 year old girl was pregnant before her father knew.
by Jennifer Hart Yim | May 12, 2015 | Big Data, Blog, Data/Analytics, Strategy
This article is part of a series of articles written by MBA students and graduates from the University of New Hampshire Peter T. Paul College of Business and Economics.
Josh Hutchins received his B.S. in Business Administration from the University of New Hampshire in 2005. He is currently pursuing his MBA at the Peter T. Paul School at the University of New Hampshire; on course to graduate in May 2016.
“Working with data is something I do every day as a financial analyst. I enjoy crunching the data and experimenting with various data analytic techniques. I’ve found that my love for playing with the data and thinking in unconventional ways has led me to be efficient and successful at work. The way data is being used is revolutionizing the way we do business. I’m glad I can be part of this wave of the future.”
The responsibility of big data
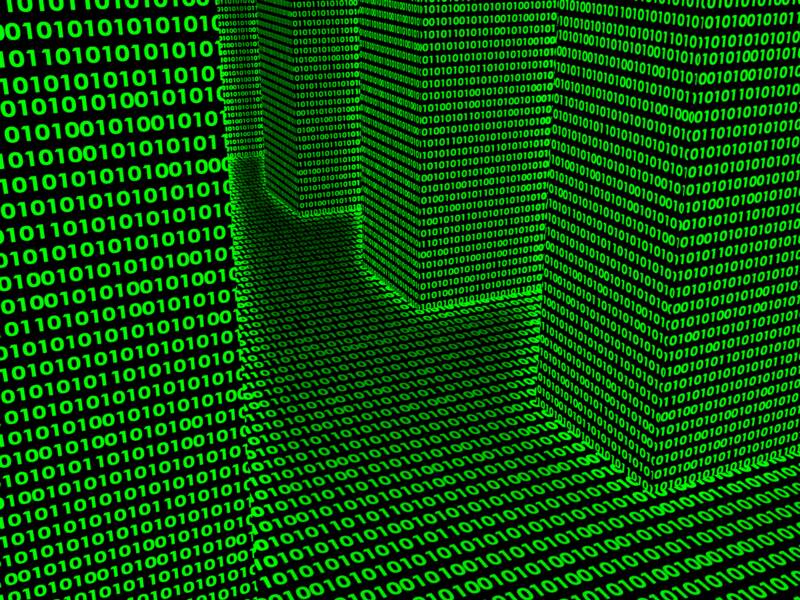
Data is coming into businesses at incredible speeds, in large quantities, and in all types of different formats. In a world full of big data it’s not just having the data – it’s what you do with it that matters. Big data analysis is becoming a very powerful tool used by companies of all sizes. Companies are analyzing and using the data in order to create sustainable business models and gain a competitive advantage over their competition. However, as one company would come to learn – with big data comes big responsibility.
Solid Gold Bomb T-Shirt Company
In 2011, Solid Gold Bomb, an Amazon Marketplace merchant based out of Australia, thought of an ingenious way to create fresh slogans for t-shirts. The main concept behind Solid Gold Bomb’s operation was that by utilizing a computer programming script, they could create clever t-shirt slogans that no one had thought of previously. The company created various t-shirt slogans that played off of the popular British WWII era phrase Keep Calm and Carry On. Under the systematic script method, Solid Gold Bombs was able to create literally millions of t-shirt offerings without the need to have them on hand in inventory. With the substantial increase in product offerings, the chances of customers stumbling upon a Solid Gold Bomb shirt increased dramatically. By utilizing this new on-demand approach to t-shirt printing, Solid Gold Bomb was able to reduce expenses, while simultaneously increasing potential revenue by offering exponential products at little additional marginal cost.
Use of ‘Big Data’
The computer script relied on the following to operate: a large pool of words, associated rule learning, and an algorithm.
Large Pool of Words – Solid Gold Bomb gathered a list of approximately 202,000 words that could be found in the dictionary. Of these words, they whittled it down to approximately 1,100 of the most popular words. Some of the words were too long to be included on a t-shirt, so the list was further culled. They settled on 700 different verbs and corresponding pronouns. These words would be used by the computer script to generate t-shirt slogans.
Associated rule learning – Associated rule learning is the degree to which two variables in a given list relate to each other. The first step in associated rule learning is to identify and isolate the most frequent variables. The second step is forming rules based on different constraints on the variables – assigning an “interestingness” factor. In the case of Solid Gold Bomb, the associated rule learning assigned an interestingness factor to verb-pronoun combinations.
Algorithm – The algorithm designed by Solid Gold Bomb was very simple. Each shirt would begin with “Keep calm and”. The algorithm script would then search through the word pool and pull back the most highly associated verb and pronoun combinations. The words would then be put into the typical format of the Keep Calm and Carry On. An image of each individual combination would be mocked up and posted to their Amazon merchant account. The process would continuously loop, creating millions of combinations.
The Big Data Blues
With one innocent mistake, Solid Gold Bomb fell apart in the blink of an eye. Amazon started getting complaints about offensive slogans on Solid Gold Bomb’s t-shirts. Images of t-shirts with phrases such as “Keep Calm and Rape Her” and “Keep Calm and Hit Her” were being sold on their Amazon merchant account. Their typical weekend orders for around 800 shirts were reduced to just 3 – few enough to count on one hand. Amazon ended up pulling their entire line of clothing, essentially putting Solid Gold Bomb out of business.
What went wrong?
While Solid Gold Bomb had a good handle on how to use data that they had gathered and how to use it to their advantage, they neglected to consider the potential hidden consequences of unintentional misuse of the data. When culling 202,000 words down to 700 useable words, words such as “rape” should have been eliminated from the useable word pool. From a high level perspective, the human mind is incapable of naming all the potential combinations of the 700 useable words without the assistance of a computer program. However, the end user needs to be aware that the computer program logic will create every potential combination based on the word pool.
Moral of the Story
Big data by itself is not beneficial to a company. The real value is in the analytics that are applied to the data. The results of the analytics can be utilized in numerous ways – to make more informed decisions, create new revenue streams, and create competitive advantages, to name a few. When a company makes the decision to utilize big data analytics, each process needs to be mapped out to have an intimate understanding of how the data will be used. In the case of Solid Gold Bomb, they failed to have this intimate understanding of how the data would be used throughout the process. As a result, they paid the ultimate price; they were not able to sustain themselves through this debacle. The morale of the story: With big data comes big responsibility. Know your data and know the potential uses of the data better. Don’t be afraid to think outside the box, but know the potential consequences.
For information about another big data faux pas, learn how Target predicted that a 16 year old girl was pregnant before her father knew.