by Fronetics | Oct 26, 2016 | Big Data, Blog, Content Marketing, Data/Analytics, Marketing
Big-data insights can help you segment your email database to better target prospects based on where they are in the buyers’ journey.
Most companies these days are swimming in a sea of big data, the great swaths of information they’ve amassed from sales records, social media connections, website leads and contacts, and online analytics.
At first glance it’s a tangle of information that is hard to organize and even harder to learn anything from. That’s a stumbling block that forward-looking businesses need to overcome. Big data can help breathe new life into one of the most reliable yet shopworn tools of the trade: email campaigns.
Embrace Big Data
A study by the executive head-hunting firm Spencer Stuart surveyed 171 companies regarding big-data usage. Just a little over half of the companies used their big data to help guide email, SEO, and SMS marketing campaigns. That’s a fairly low rate, given the potential leg-up that big data can provide.
Consider what Walmart is doing. The company has big-data information on about 60% of all Americans, with which it micro-targets customers based on their individual interests and habits. It’s a powerful strategy that is spreading quickly to businesses of all sizes.
How can you use big data to freshen up your email campaigns?
Be a Collector, Not a Hoarder
Chances are, you are obtaining a lot of data, especially if you have an active content marketing plan in place. Not all of the data you get is equally important. Your focus should be on data that can lead to an actionable and quick response — for example, are you gathering information on your customers’ buying habits? Do you know who they are, where they are, what their interests are, what their email address is, and how your business connects with them?
Collect that relevant data and study it. Much of it will come from the buyer’s journey — the breadcrumbs that potential buyers leave for you in your big data. These pieces of information are keys to your personalized email responses.
Respond In Kind
Most experts agree that a quick and targeted email response is a good strategy for encouraging a new customer to make a purchase. The email needs to respond directly to the buyer’s interests — using information you’ve (hopefully) logged with your big data.
From this point on, it’s crucial to make sure that every email that is sent to that buyer is built around a backbone of big data. Nurture your customers with personalized emails that offer content and deals that line up with their specific interests.
Don’t Mess with the Masses
Mass emails — the generic sales pitch email — used to be the cost-effective and simple way of reaching and converting customers. Now, it’s more than likely they’ll get sent to the trash, or worse, the spam filter. The mass email is your one-way ticket to spam purgatory.
“Traditional methods of mass marketing doesn’t resonate anymore and they’re being ignored by the audience,” said Volker Hildebrand, Global Vice President of Strategy at SAP Hybris, in a recent interview with Forbes. “Data is the fuel for customer engagement, and being able to pull together all the relevant information about in real-time.”
You can do better than the mass email approach. If you’ve collected relevant data and you’ve studied your buyers’ journeys, you have the tools in place to build a smart email campaign. Tailor your campaign to personalize your approach to your customers, and more than likely they’ll open that email.
Related posts:
by Fronetics | Oct 20, 2016 | Big Data, Blog, Data/Analytics, Logistics, Manufacturing & Distribution, Strategy, Supply Chain, Warehousing & Materials Handling
These industry leaders are leveraging insights from big data to solve business problems and drive profitable customer action.
Big data is more than just a buzzword: It’s helping companies make big-impact business decisions based on customer behaviors, purchasing patterns, and preferences.
Not all organizations have the resources to invest in big data. But for those that do, the payoff can big significant. The trick, of course, is knowing which numbers to analyze, what can be predicted, how to use big data for your particular business needs.
Let’s look at five big brands that are leveraging big data successfully to drive profitable customer action.
How 5 big brands use big data
1. Amazon
Amazon’s free same-day delivery service, Prime Now, allows Prime members to shop for over 25,000 products that can be delivered to their doorsteps within two hours. How can Amazon accurately predict the specific wants of millions of people across the country at a given time to prepare local inventory for immediate delivery? Aside from efficient warehousing and logistics, the company uses data on purchase history to optimally locate and stock its warehouses. This strategy also helps to reduce the time inventory stays in stock, diminishing working capital requirement.
2. Starbucks
Have you ever wondered how two neighborhood Starbucks locations can both stay in business? The company examines data on local traffic, demographics, and customers to determine the potential success of a new location before expanding. Starbucks can then choose to open a new store where it would be most successful, even if it’s just a few blocks from one of their other locations.
3. Walmart
In preparation for Hurricane Sandy, Walmart analyzed historical sales data before expected inclement weather and found an uptick in sales of flashlights, emergency equipment, and — to everyone’s surprise — strawberry Pop-Tarts in several locations. The company has since leveraged timely analysis of real-time data to drive business performance. As Walmart Senior Statistical Analyst Naveen Peddamail told Forbes: “If you can’t get insights until you’ve analysed your sales for a week or a month, then you’ve lost sales within that time. Our goal is always to get information to our business partners as fast as we can, so they can take action and cut down the turnaround time. It is proactive and reactive analytics.”
4. Rolls-Royce
Rolls-Royce has implemented big data processes in three key areas of their operations: design, manufacture, and after-sales support. Each design simulation for one of their jet engines, for example, generates tens of terabytes of data, which computer systems analyze to determine the viability of the design. The company’s manufacturing systems are increasingly moving toward a networked, Internet of Things (IoT) industrial environment. And after-sales support is completely changed by big-data analysis. Expert engineers continually examine real-time analysis from sensors fitted to all Rolls-Royce engines and propulsion systems to diagnose faults and mitigate issues.
5. Capital One
Big data helps Capital One determine the optimal times to send particular customers certain offers. The team analyzes demographic data and spending habits of their customers to optimize their offerings, which has increased conversion rates on their offers and generated more leads from their marketing budget.
How does your business use big data?
Related posts:
by Fronetics | Sep 7, 2016 | Big Data, Blog, Data/Analytics, Logistics, Strategy, Supply Chain
While the temptation to invest in big data may be strong, small and mid-sized companies might be better off starting with small, more manageable analytics.
Big data has become a big business. A 2016 Forrester report forecasts that the big-data management business will grow by 12.8% by 2021. It’s easy to see why, with the prevalence of stories from brands like Wamart and Rolls Royce that have perfected the science of capitalizing on detailed insights about customer behavior.
But many organizations — particularly small to mid-sized companies — are learning that big data does not always equate to big payoffs. In fact, many organizations have sunk millions of dollars into sophisticated data-analytics software only to realize they don’t have the capabilities to interpret the new insights nor the expertise to turn them into a competitive advantage.
Big data and the supply chain
Companies within the logistics and supply chain industries aren’t immune to this trend. An Accenture survey of more than 1,000 supply chain executives found that 84% have already employed big-data analytics or are in the latter stages of planning to do so. Respondents reported:
- 17% have implemented analytics in one or more supply chain functions.
- Three out of 10 have an active initiative to implement analytics in 6-12 months.
- More than one-third (37%) were engaged in serious conversations about implementation.
The promising applications of big data and how it might “revolutionize” the supply chain are hard to ignore. Increased efficiency across the supply chain, improved forecasting, better cost control, more accurate inventory planning: the list of benefits go on and on. These could lead to a significant advantage for companies with the expertise, structure, and knowledge to collect, analyze, and draw strategy cues from large sets of raw data.
But, again, small and mid-sized companies usually aren’t well positioned to do so, unfortunately.
Instead, start small
Supply chain and logistics organizations that don’t have such capabilities — or the capital to invest in technology that can do it for them — should start small instead. Even if you eventually want to implement a big-data strategy, there is a long-term benefit to gleaning insight from small data sets in the interim.
Here are some steps for getting started:
1) Determine your goals
Consider your business objectives and how you can leverage data to further your goals. Focus on just one or two areas for improvement, and clearly articulate what kinds of data you want to collect as they relate to those objectives.
2) Identify your sources
From where will you collect your data? Analyzing numerous data sets from a variety of sources will leave your head spinning and you none the wiser. Also determine which metrics will be most helpful to you, and which you can set aside.
3) Pick your people
If your company doesn’t have in-house analytics expertise, work to attract the appropriate talent. Regardless, integration and structuring of analytic personnel positions will be a more significant factor in your success than your use of even the most advanced statistical software program.
4) Master reporting
Finally, spend some time determining how your findings should be presented. You’ll want them to be formatted in a clear, digestible manner with a clear application for how they will improve your business.
Related posts:
by Fronetics | Jan 14, 2016 | Big Data, Blog, Data/Analytics, Strategy, Supply Chain
Fronetics Strategic Advisors is a leading management consulting firm. We advise and work with companies on their most critical issues and opportunities: strategy, marketing, organization, talent acquisition, performance management, and M&A support.
We’ve gathered our most-read big data articles of 2015:
With current technologies, there are increasing amounts of information to be gathered and exchanged in the world, creating more opportunities for businesses to harness that information and chart a course, or to tweak processes based on that information. According to an Accenture study, “97% of executives report having an understanding of how Big Data analytics can benefit their supply chain, but only 17% said that they have implemented it in at least one supply chain function.” Read the full article.
KPMG recently conducted a survey of 144 CFOs and CIOs with the objective of gaining a more concrete understanding of the opportunities and challenges that big data and analytics present. The survey found that 99% of respondents believe that data and analytics are at least somewhat important to their business strategies; and 69% consider them to be crucially or very important. Despite the perceived value of big data, 85% of respondents reported that they don’t know to analyze and interpret the data they already have in hand (much less what to do with forthcoming data). Read the full article.
Forget for a moment the potential of adopting big data analytics throughout the entire supply chain, and consider instead how big data can untangle and integrate seemingly unrelated masses of data to solve small problems in a warehouse or distribution center. That’s exactly what this company did. Read the full article.
Executives are eager to jump on the bandwagon, too. Although only 13% of 250 executives surveyed by Accenture said they use big data primarily for predictive purposes, as many as 88% indicated big data analytics is a top priority for their company. With an increasing number of companies learning to master the precursors to developing predictive models — namely, connecting, monitoring, and analyzing — we can safely assume the art of gleaning business intelligence from foresight will continue to grow. Read the full article.
by Fronetics | Sep 3, 2015 | Big Data, Blog, Data/Analytics, Logistics, Strategy, Supply Chain
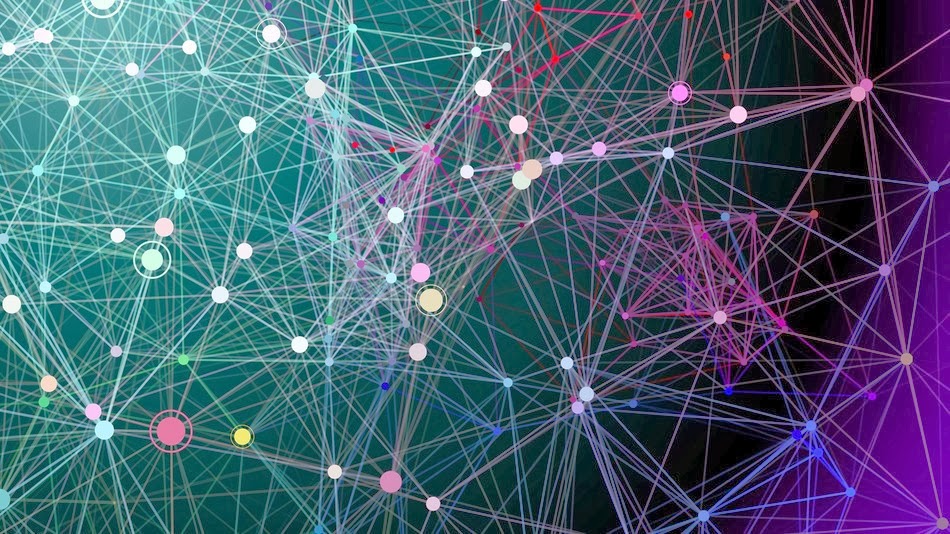
Big data is, well, big. The term has gotten lots of buzz the past few years. But it’s big in other ways as well. According to McKinsey big data is defined as “datasets whose size is beyond the ability of typical database software tools to capture, store, manage, and analyze.” A 2014 Forbes article has 11 other helpful definitions.
With current technologies, there are increasing amounts of information to be gathered and exchanged in the world, creating more opportunities for businesses to harness that information and chart a course or tweak processes based on that information. According to an Accenture study, “97% of executives report having an understanding of how Big Data analytics can benefit their supply chain, but only 17% said that they have implemented it in at least one supply chain function.”
In a Boston Consulting Group article, Making Big Data Work: Supply Chain Management, the authors suggest three high-potential opportunities for supply chain management to take advantage of big data. Their suggestions help parse through the complicated, overwhelming network of big data. As they suggest, “with so much available data and so many improvable processes, it can be challenging for executives to determine where they should focus their limited time and resources.” Let’s have a look at the authors’ ideas, as gathered through research, on how to “increase asset uptime and expand throughout, engage in preventive maintenance of production assets and installed products, and conduct near real-time supply planning using dynamic data feeds from production sensors and the Internet of Things.”
Visualizing Delivery Routes
Big data, in the form of geoanalytics, can be used to better manage supply chain routes and help reduce transportation costs by 15-20%, especially when other partner companies are trying to coordinate deliveries. “The companies learned that they shared similar patterns of demand. Vehicle-routing software also enabled rapid scenario testing of dozens of route iterations and the development of individual routes for each truck. Scenario testing helped the companies discover as much as three hours of unused delivery capacity on typical routes after drivers had covered their assigned miles.” Real-time data from live traffic feeds, combined with past data helped to create new forecasts and eliminate wasted time.
Pinpointing Future Demand
In today’s volatile marketplace, relying on sales predictions can be risky and inaccurate, at best, a disaster at worst. According to the BCG article, advanced languages can now work together to create a most accurate forecast, freeing up sales people and their precious time to combat other issues and convert leads. “Advanced analytical techniques can be used to integrate data from a number of systems that speak different languages—for example, enterprise resource planning, pricing, and competitive-intelligence systems—to allow managers a view of things they couldn’t see in the past.”
Simplifying Distribution Networks
The European consumer goods company profiled in the BCG article, used advanced analytics to downsize from 80 factories across 10 countries, down to 20 factories. The distribution network shrunk, and efficiency and savings increased, the latter by 8%. Working with big data can help examine a diverse amount of information never before analyzed. Taking complex data and knowing how to handle the data can turn complexity into simplicity.
Marcelo Simiao, Lean Manager— Operations at Munters, seems to concur with the findings, and believes big data can make sweeping changes.
“Companies are struggling with today’s greater demand volatility. The Order-to-Delivery processes have been the focus of many improvement projects and lean initiatives that aim to reduce costs and improve response times. But most of today’s organizations have their supply chain functions fragmented into several different departments, creating process improvement projects that have results limited to the data they have at hand. This approach often doesn’t deliver value to the end customer. Big Data is changing this scenario by integrating the voice of the customer, sales and the entire supply chain. This integration and thorough data analysis allows organizations to align all of their focus on key projects that are not limited by functions, will improve customer satisfaction, and deliver results directly into the company’s bottom line.”
by Elizabeth Hines | Sep 1, 2015 | Big Data, Blog, Data/Analytics, Strategy, Supply Chain
Many organizations that jumped on the big data bandwagon have struggled to turn their new, boundless collection of data into actionable business information. As consultant Rich Sherman of Athena IT Solutions puts it, today’s businesses are struggling with “the transformation of data into information that is comprehensive, consistent, correct and current.”
Enter data governance programs, and, consequently, the data steward. Technology cannot always extract the most useful data, but the data stewards can, meaning the success of the data governance program rests to a large degree on their shoulders. But data stewards can be viewed with suspicion by other employees and nothing can kill the spirit of hard work more than distrust or a feeling that management is snooping and ready to pounce at the sign of even the slightest misstep.
Some business users are under the impression data stewards have been tasked to play dual roles, championing the organization’s data governance program while also using their position to crack down on anyone stepping out of line. To some, the data steward is really a data cop who “police” rather than manage the organization’s critical data elements. They believe anyone can be caught in the net cast by the data stewards as they fish for out-of-compliance data and bring it into line with policy or regulatory obligations. This can create needless friction within the organization and threaten the effectiveness of data efforts altogether. We can’t ignore concerns caused by the growing presence of data stewards at many organizations; in fact, it makes it even more important to show why such concerns are generally unfounded.
While it is true data stewards are indeed tasked with ensuring compliance with the policies and processes of the data governance program, critics need to bear the end goal in mind – to turn massive amounts of data into a useable corporate asset. And data stewards themselves can actually play a role in the effort to help “to take the view of data governance from police action to harmonious collaboration,” as another expert, Anne Marie Smith of Alabama Yankee Systems, LLC, put it. It won’t hurt for the data stewards – or the data governance managers – to acknowledge some employees will initially question their intentions. However, by reaching out to each business unit and explaining how data governance works and why improved data management will benefit the organization, the distrust can dissipate. Similarly, if the data steward is recruited from within the organization, it will alleviate some concerns since business users are more likely to trust a familiar face.
The complexity of data governance comes with a host of pitfalls – fears of data cops shouldn’t be one of them. What’s been your experience with data stewards in the supply chain? Do they play an important role in your organization?