by Jennifer Hart Yim | May 18, 2015 | Blog, Logistics, Strategy, Supply Chain
This article is part of a series of articles written by MBA students and graduates from the University of New Hampshire Peter T. Paul College of Business and Economics.
Michael Hickey is a former fifth grade teacher turned business professional. His experience includes content marketing in the IT industry and operations management for United Parcel Service. He will complete his MBA from the University of New Hampshire in 2015. He enjoys long walks along the conveyor belt and Ben and Jerry’s ice cream. He lives with his wife, Betsy, in Dover, New Hampshire and they are expecting their first child in June.
4 questions to ask when determining if a 3PL is right for your company
Third-party logistics, or 3PL, is an industry on the rise thanks to the constant innovations in complementary industries like telecommunications, data analytics, and cloud technologies. To avoid confusion, let’s call 3PL what it is: outsourcing. But it’s not the kind of outsourcing that typically comes to mind when you hear the term. Rather, it’s a specific type of outsourcing related to the operations side of a company in areas like order fulfillment, inventory and warehouse management, or transportation of finished goods. As many companies, and perhaps your competitors, begin to employ some form of 3PL, you may be tempted to follow suit. But before you hand over the keys, consider whether or not 3PL is a good fit for your company by answering these four questions:
Question 1: What are your company’s core values?
Why do you exist as a company? What service or product do you provide that you believe is better than all others like it? And what are the core values that your company adheres to in good times and bad, for better or for worse? Core values make you who you are. They are the DNA of the company. Stonyfield Farm, for example, produces a variety of yogurts from their New Hampshire-based facility. One of their core values is that they use only organic ingredients, sourced from family-owned organic farms in their products. No ifs, ands, or buts. That’s a core value. It won’t change, during either boom or recession. And everything they do as a company must align with that. Your company’s strategic alignment stems from identification of its core values, and each decision you make as a company should work seamlessly with your strategic alignment.
Action step: Identify your core values. If 3PL conflicts with any core values, you should avoid forcing its implementation, even if there are cost savings to be gained.
Question 2: What are your company’s core competencies?
What are the things that your company does well? The Yankee Candle Company’s core competencies lie within their research and development and the chemists they employ. Their specialized skills and olfactory expertise drive the creation of precisely scented candles that make you say, “I know I smell a pumpkin pie, but I can’t find it anywhere!” Their competencies help them stand apart from the competition. You would be remiss to give over your core competency to someone else. If your expertise lies in local delivery and timely service, why outsource it to the guys with the brown trucks?
Action step: Identify your core competencies. If 3PL takes the place of any part of your core competencies, you could be weakening the overall value proposition of your company.
Question 3: Will using a 3PL provider allow you to enhance your core competencies to meet your company’s goals?
The purpose of debating whether or not to employ 3PL providers should not focus so much on reduced costs, which can be one of the foremost benefits, but rather whether or not it can enhance your core competencies and stimulate growth for your company. Is your goal to reach broader markets, but you lack the expertise to make it happen? Perhaps an e-commerce fulfillment provider could help you reach those markets. Do you have an outstanding product, but can only sell it to those within a small radius of your operations? Maybe this would be the appropriate time to call on the guys with the brown trucks.
Action step: Draw parallels between the service you wish to outsource and the goal it will meet.
Question 4: What is the cost to your company?
It’s the question that always needs to be considered. But don’t take this question at face value: we’re not just talking about how choosing a 3PL will affect the bottom line. Of course there will be monetary costs associated with hiring another company, and there is even a tipping point when using a 3PL may be cost ineffective. So after a careful cost/benefit analysis, consider the other costs associated with handing over part of your value chain to a third party:
Time costs: Does outsourcing add lead times or delivery times to orders? Decide whether possible time costs take away from your value proposition, or enable your company to meet larger goals.
Control costs: Are you willing to hand over direct control of part of your value chain to someone else? Keep in mind that it’s possible no one cares about your business quite as much as you do. Can you trust someone else to make the same kind of decisions you would make in respect to your company and its customers?
Reputation costs: What happens if a 3PL provider does not perform as anticipated? Will it put a blemish on your company’s image? If a farm outsources its delivery to a local trucking company, and the refrigeration in the trucks falters and causes food to spoil, will the customer assume that the trucking was bad, or do they just assume that the quality of the produce from the farm is questionable? It takes a long time to build up a reputation, and only a short time to dismantle it. Don’t risk it on a provider you can’t trust.
Action step: Vet your possible 3PL options to see whose values closely align with yours. It may prove to be a critical step in choosing the right provider as opposed to the cheapest one.
Third party logistics provides an avenue for companies to scale to capabilities they may never have had the ability to reach. Expanded consumer markets, faster delivery times, and more efficient inventory management are some of the benefits to be had. But before you get drawn towards the soft glow of higher revenues and wider margins through outsourcing, be careful to make sure that your choice to engage 3PL providers aligns with your company’s strategic plans. And if you do choose to outsource, take your time to find the right provider who can add the most value to your business, not just the least amount of digits on the balance sheet.
by Jennifer Hart Yim | May 18, 2015 | Blog, Logistics, Strategy, Supply Chain
This article is part of a series of articles written by MBA students and graduates from the University of New Hampshire Peter T. Paul College of Business and Economics.
Michael Hickey is a former fifth grade teacher turned business professional. His experience includes content marketing in the IT industry and operations management for United Parcel Service. He will complete his MBA from the University of New Hampshire in 2015. He enjoys long walks along the conveyor belt and Ben and Jerry’s ice cream. He lives with his wife, Betsy, in Dover, New Hampshire and they are expecting their first child in June.
4 questions to ask when determining if a 3PL is right for your company
Third-party logistics, or 3PL, is an industry on the rise thanks to the constant innovations in complementary industries like telecommunications, data analytics, and cloud technologies. To avoid confusion, let’s call 3PL what it is: outsourcing. But it’s not the kind of outsourcing that typically comes to mind when you hear the term. Rather, it’s a specific type of outsourcing related to the operations side of a company in areas like order fulfillment, inventory and warehouse management, or transportation of finished goods. As many companies, and perhaps your competitors, begin to employ some form of 3PL, you may be tempted to follow suit. But before you hand over the keys, consider whether or not 3PL is a good fit for your company by answering these four questions:
Question 1: What are your company’s core values?
Why do you exist as a company? What service or product do you provide that you believe is better than all others like it? And what are the core values that your company adheres to in good times and bad, for better or for worse? Core values make you who you are. They are the DNA of the company. Stonyfield Farm, for example, produces a variety of yogurts from their New Hampshire-based facility. One of their core values is that they use only organic ingredients, sourced from family-owned organic farms in their products. No ifs, ands, or buts. That’s a core value. It won’t change, during either boom or recession. And everything they do as a company must align with that. Your company’s strategic alignment stems from identification of its core values, and each decision you make as a company should work seamlessly with your strategic alignment.
Action step: Identify your core values. If 3PL conflicts with any core values, you should avoid forcing its implementation, even if there are cost savings to be gained.
Question 2: What are your company’s core competencies?
What are the things that your company does well? The Yankee Candle Company’s core competencies lie within their research and development and the chemists they employ. Their specialized skills and olfactory expertise drive the creation of precisely scented candles that make you say, “I know I smell a pumpkin pie, but I can’t find it anywhere!” Their competencies help them stand apart from the competition. You would be remiss to give over your core competency to someone else. If your expertise lies in local delivery and timely service, why outsource it to the guys with the brown trucks?
Action step: Identify your core competencies. If 3PL takes the place of any part of your core competencies, you could be weakening the overall value proposition of your company.
Question 3: Will using a 3PL provider allow you to enhance your core competencies to meet your company’s goals?
The purpose of debating whether or not to employ 3PL providers should not focus so much on reduced costs, which can be one of the foremost benefits, but rather whether or not it can enhance your core competencies and stimulate growth for your company. Is your goal to reach broader markets, but you lack the expertise to make it happen? Perhaps an e-commerce fulfillment provider could help you reach those markets. Do you have an outstanding product, but can only sell it to those within a small radius of your operations? Maybe this would be the appropriate time to call on the guys with the brown trucks.
Action step: Draw parallels between the service you wish to outsource and the goal it will meet.
Question 4: What is the cost to your company?
It’s the question that always needs to be considered. But don’t take this question at face value: we’re not just talking about how choosing a 3PL will affect the bottom line. Of course there will be monetary costs associated with hiring another company, and there is even a tipping point when using a 3PL may be cost ineffective. So after a careful cost/benefit analysis, consider the other costs associated with handing over part of your value chain to a third party:
Time costs: Does outsourcing add lead times or delivery times to orders? Decide whether possible time costs take away from your value proposition, or enable your company to meet larger goals.
Control costs: Are you willing to hand over direct control of part of your value chain to someone else? Keep in mind that it’s possible no one cares about your business quite as much as you do. Can you trust someone else to make the same kind of decisions you would make in respect to your company and its customers?
Reputation costs: What happens if a 3PL provider does not perform as anticipated? Will it put a blemish on your company’s image? If a farm outsources its delivery to a local trucking company, and the refrigeration in the trucks falters and causes food to spoil, will the customer assume that the trucking was bad, or do they just assume that the quality of the produce from the farm is questionable? It takes a long time to build up a reputation, and only a short time to dismantle it. Don’t risk it on a provider you can’t trust.
Action step: Vet your possible 3PL options to see whose values closely align with yours. It may prove to be a critical step in choosing the right provider as opposed to the cheapest one.
Third party logistics provides an avenue for companies to scale to capabilities they may never have had the ability to reach. Expanded consumer markets, faster delivery times, and more efficient inventory management are some of the benefits to be had. But before you get drawn towards the soft glow of higher revenues and wider margins through outsourcing, be careful to make sure that your choice to engage 3PL providers aligns with your company’s strategic plans. And if you do choose to outsource, take your time to find the right provider who can add the most value to your business, not just the least amount of digits on the balance sheet.
by Jennifer Hart Yim | May 14, 2015 | Blog, Strategy, Supply Chain
This article is part of a series of articles written by MBA students and graduates from the University of New Hampshire Peter T. Paul College of Business and Economics.
Sarah Hebert is currently a full-time MBA student at the University of New Hampshire, having previously completed her Bachelor’s of English Language and Literature at the same institution in 2014. Following the completion of her Master’s degree, she hopes to find a job in Marketing and Advertising.
When “Food with Integrity”meets the bottom-line.
As Chipotle’s nation-wide gastronomic success has propelled their brand into the fast-casual limelight, so followed the news of their carnitas crisis, as the chain announced in January 2015 that they were and would be experiencing a significant strain in their Responsibly Raised® Pork supply for the foreseeable future.
Chipotle®, a Mexican fast-casual restaurant chain that has built its brand on self-established food quality standards, such as organic, local vegetables and ethically raised animals, made waves with their voluntary decision to cut their pork supply due to a supplier violation of their animal welfare standards. As stated by the chain, pigs living within these pork farms must be:
- Raised with outdoor access or deeply bedded pens
- Free of breeding, gestation, and farrowing crates
- Never treated with antibiotics
Upon discovering that this particular supplier did not allow their animals to have outdoor access and did not supply the alternative pens that were required under those conditions, Chipotle immediately ceased their business with the farm in question. As a result, 1/3 of their chain-wide supply was eliminated, directly affecting the 7-8% of total sales that are accounted for by their pork-consuming customers.
Rather than keeping mum about this potentially destructive development, Chipotle defied expectations and proactively embraced this supply-chain debacle as a strategic PR opportunity, fully acknowledging their decision and citing their core values as their motive. On the front end of their business, Chipotle addressed this issue across all channels—attention grabbing signage on storefronts, disclaimers at the launch of their online ordering services, an FAQ page on their website solely dedicated to their carnitas shortage, and countless press releases provided to news sources across the country.
On the back end, the chain exercised several strategies to try to compensate for the consequences of their actions. In a short-term attempt to alleviate the additional, compensatory pressures faced by their remaining pork suppliers and to satisfy pork-consuming customers where possible, Chipotle chose to geographically rotate their carnitas supplies around the country every 6 weeks. Realizing that their characteristically high standards for food sourcing would continue to be tested as the chain continues to grow at an astronomical pace (currently operating approximately 1800 locations nation-wide), Chipotle wasted no time to begin addressing their long-term supply concerns, exploring solutions such as acquiring additional suppliers, integrating new cuts of pork into their carnitas option, and feasibly increasing the amount of meat secured from their current providers in order to best insulate themselves from future supply constraints.
Since their notorious announcement back in January, it is estimated the Chipotle’s decision to stand by their ethically-poised brand and diminish their supplies volume will cost them nearly a year of operating at full capacity, projecting that the chain’s shortage will not completely recover until the end of Q4. Translation? No carnitas until December 2015. At the conclusion of the first quarter of 2015, Chipotle fell 1.3% short of their projected 11.7% sales growth put forth by Wall Street, achieving a growth rate of only 10.4% total.
Despite Chipotle’s applaud-worthy success at capitalizing on this marketing opportunity by means of reinforcing their “Food with Integrity” adage and raising awareness for animal welfare, the question shifts from a concern regarding their recovery timeline to one of sustainability and future growth. As the chain continues to mature and prosper, are their sourcing practices and business model sustainable? At what point do you scale back your growth for the sake of maintaining your brand integrity, or to better meet your demand with an already constrained supply? While the admirable decision made by the chain played nicely into the hands of their marketing and public relations teams, one has to wonder about how these decisions may jeopardize the future of their consumer dependability–ultimately, at what cost is your pride more important than your profits?
To say that Chipotle turned the tides on their self-inflicted disaster would be an understatement—more than ever, the chain is being commended for their devotion to their ethical standards and continues to gain national attention for their damage control tactics. As the problem has yet to be completely rectified and the light is still being brightly shone on Chipotle’s radical supply-chain stance, the eyes of the consumers remain locked on the positive statement being made in the here and now. After the storm passes and carnitas are back in the hands of Chipotle customers, the reliability and sustainability of their sourcing practices will undoubtedly come into question. With growth of business comes increased demand, and as the company has yet to establish a long-term supply solution for its Responsibly Raised meats, it’s certainly not irrational to wonder if the fast-casual kingpin will be as lucky to weather the storm the next time around.
by Jennifer Hart Yim | May 14, 2015 | Blog, Strategy, Supply Chain
This article is part of a series of articles written by MBA students and graduates from the University of New Hampshire Peter T. Paul College of Business and Economics.
Sarah Hebert is currently a full-time MBA student at the University of New Hampshire, having previously completed her Bachelor’s of English Language and Literature at the same institution in 2014. Following the completion of her Master’s degree, she hopes to find a job in Marketing and Advertising.
When “Food with Integrity”meets the bottom-line.
As Chipotle’s nation-wide gastronomic success has propelled their brand into the fast-casual limelight, so followed the news of their carnitas crisis, as the chain announced in January 2015 that they were and would be experiencing a significant strain in their Responsibly Raised® Pork supply for the foreseeable future.
Chipotle®, a Mexican fast-casual restaurant chain that has built its brand on self-established food quality standards, such as organic, local vegetables and ethically raised animals, made waves with their voluntary decision to cut their pork supply due to a supplier violation of their animal welfare standards. As stated by the chain, pigs living within these pork farms must be:
- Raised with outdoor access or deeply bedded pens
- Free of breeding, gestation, and farrowing crates
- Never treated with antibiotics
Upon discovering that this particular supplier did not allow their animals to have outdoor access and did not supply the alternative pens that were required under those conditions, Chipotle immediately ceased their business with the farm in question. As a result, 1/3 of their chain-wide supply was eliminated, directly affecting the 7-8% of total sales that are accounted for by their pork-consuming customers.
Rather than keeping mum about this potentially destructive development, Chipotle defied expectations and proactively embraced this supply-chain debacle as a strategic PR opportunity, fully acknowledging their decision and citing their core values as their motive. On the front end of their business, Chipotle addressed this issue across all channels—attention grabbing signage on storefronts, disclaimers at the launch of their online ordering services, an FAQ page on their website solely dedicated to their carnitas shortage, and countless press releases provided to news sources across the country.
On the back end, the chain exercised several strategies to try to compensate for the consequences of their actions. In a short-term attempt to alleviate the additional, compensatory pressures faced by their remaining pork suppliers and to satisfy pork-consuming customers where possible, Chipotle chose to geographically rotate their carnitas supplies around the country every 6 weeks. Realizing that their characteristically high standards for food sourcing would continue to be tested as the chain continues to grow at an astronomical pace (currently operating approximately 1800 locations nation-wide), Chipotle wasted no time to begin addressing their long-term supply concerns, exploring solutions such as acquiring additional suppliers, integrating new cuts of pork into their carnitas option, and feasibly increasing the amount of meat secured from their current providers in order to best insulate themselves from future supply constraints.
Since their notorious announcement back in January, it is estimated the Chipotle’s decision to stand by their ethically-poised brand and diminish their supplies volume will cost them nearly a year of operating at full capacity, projecting that the chain’s shortage will not completely recover until the end of Q4. Translation? No carnitas until December 2015. At the conclusion of the first quarter of 2015, Chipotle fell 1.3% short of their projected 11.7% sales growth put forth by Wall Street, achieving a growth rate of only 10.4% total.
Despite Chipotle’s applaud-worthy success at capitalizing on this marketing opportunity by means of reinforcing their “Food with Integrity” adage and raising awareness for animal welfare, the question shifts from a concern regarding their recovery timeline to one of sustainability and future growth. As the chain continues to mature and prosper, are their sourcing practices and business model sustainable? At what point do you scale back your growth for the sake of maintaining your brand integrity, or to better meet your demand with an already constrained supply? While the admirable decision made by the chain played nicely into the hands of their marketing and public relations teams, one has to wonder about how these decisions may jeopardize the future of their consumer dependability–ultimately, at what cost is your pride more important than your profits?
To say that Chipotle turned the tides on their self-inflicted disaster would be an understatement—more than ever, the chain is being commended for their devotion to their ethical standards and continues to gain national attention for their damage control tactics. As the problem has yet to be completely rectified and the light is still being brightly shone on Chipotle’s radical supply-chain stance, the eyes of the consumers remain locked on the positive statement being made in the here and now. After the storm passes and carnitas are back in the hands of Chipotle customers, the reliability and sustainability of their sourcing practices will undoubtedly come into question. With growth of business comes increased demand, and as the company has yet to establish a long-term supply solution for its Responsibly Raised meats, it’s certainly not irrational to wonder if the fast-casual kingpin will be as lucky to weather the storm the next time around.
by Jennifer Hart Yim | May 13, 2015 | Blog, Leadership, Strategy, Supply Chain
This article is part of a series of articles written by MBA students and graduates from the University of New Hampshire Peter T. Paul College of Business and Economics.
Corey Ducharme is Green Belt Certified in Six Sigma. He has a BA in Business and is currently in the MBA program at the University of New Hampshire. He has consulted at major corporations including Johnson & Johnson, Universal Studios, Sony Pictures, Oklahoma Oil & Gas, and Suncor as a management consultant at D&A Management. He can be reached via e-mail.
We all use Six Sigma problem solving whether we know it or not
How do humans tend to solve problems? Consciously or unconsciously humans use a four-step method that is defined as:
Table 1: Traditional Problem Solving Process

In the above example, the root cause is identified – exercise and eating less equals losing weight – and verified. The conventional wisdom is proven true and there is little need to consider a more robust or analytical method. Most humans solve their day-to-day issues in this manner whether consciously or unconsciously. My root-cause analysis in this case (I exercise and eat less) is verified by the fact that I lost ten pounds and sustainable until my goal is achieved. (Losing 20 pounds).
Six Sigma Problem Solving 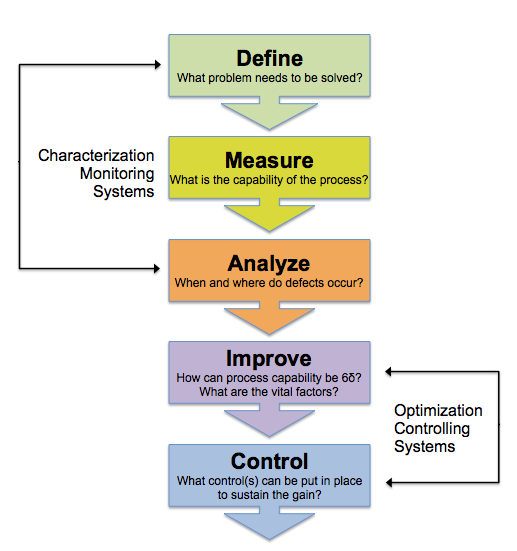
What if the root-cause lies outside of conventional wisdom or is difficult to determine?
These needle-in-a-haystack problems – due to limited business resources – cause businesses to lose revenue and lead to process failures, poor quality, and poor customer service. These types of problems are at the heart of Six Sigma Problem Solving and are a way to find the needle-in-the-haystack.
The Six Sigma methodology is based on the DMAIC process and using our weight loss example we begin to see the similarities between the two methods.
- Define Phase: What is the problem and set the end goal.
- Measure Phase: What is the current state?
- Analyze Phase: 1. Develop cause-and-effect analysis of problem. What are the real causes and prove cause and effect links.
- Improve Phase: Action
- Control Phase: 1. Verify improvement and 2. Sustainability
What differs between the phases of conventional and Six Sigma problem solving begins in the Analyze Phase. Six Sigma methodology demands the proof of cause be determined before a clear course of action is taken. The proof of cause must be data-driven as root-cause analysis is at the core of Six Sigma problem solving. It is also the reason that the Analyze phase is divided into the development of cause-effects and proof of cause-effect links.
The second difference is in the fifth phase. In the Traditional problem-solving methodology, verification comes in the form of losing weight. I can prove it by weighing myself. In Six Sigma problem solving, a two-step process is needed. Verification is essential (Did I improve?) and if so, a plan to sustain our gains is created. This is not necessary in a Traditional methodology because our cause-effect is proven (exercise/eat less = weight loss); however, in the Six Sigma methodology our cause-effect must be tested and verified.
The Linguistics of Six Sigma: Y=f(X)
To speak in the language of Six Sigma, we need to change ‘problem’ with ‘Y’ and ‘cause’ with ‘X’. The Y is the output and the X(s) are any inputs that are involved in producing the output. In other words, the Y = 100% customer satisfaction and X(s) are the variables that affect the level of customer satisfaction. For more, click the following link: Y=f(X).
Using Six Sigma linguistics and the DMAIC process, we can combine the Traditional problem solving steps in Table 1 and we see that our four-step process has become a more robust seven-step process. We can now use DMAIC to ask ourselves the most essential question:
If our root-cause analysis is discovered and proven true, then can the problem be solved or reduced by controlling or removing the cause(s)?
Table 2: DMAIC Problem Solving
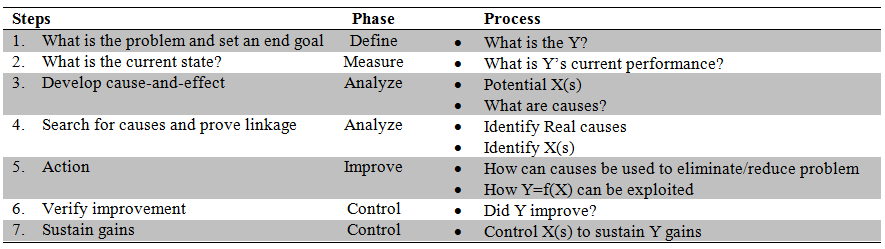
Real World Example: Boeing
Boeing’s Six Sigma team in Everett, WA discovered that root cause analysis is often like finding a needle-in-a-haystack, especially for the maker of the world’s largest commercial twin-engine airplane with millions of components. (To read the entire story, click the following link: Problem-solving approach helps team pinpoint solution). Boeing discovered that recirculating air fans were being rejected during production, costing Boeing money in waste, removal, testing, and cost of replacing the component.
Boeing assembled a cross-functional ‘detective squad’ that included employees from Engineering, Quality, Manufacturing, Supply Management, Procurement, and their supplier Hamilton Sundstrand who began the problem-solving techniques of the DMAIC process by examining data from the fans and beginning root-cause analysis.
This analysis determined that Foreign Object Debris (FOD) damaged the fans and the test tools. Job done, right? Not according to Kent Kuiper, Six Sigma expert at Boeing, the team had to dig deeper. “For example,” he said, “when we found that FOD was a problem and determined the source for it, removing the FOD and replacing the fan wasn’t going to get us where we needed to go. We had to figure out a way to keep the FOD from happening again.”
Further inspection led the team to discover failures in ductwork caps and plastic sheeting – two items ironically intended to prevent FOD damage and two electrical issues. One failure of improperly modified test equipment and the second issue related to crimping procedures in manufacturing process that improved connections in the fans. The results? Although the FOD in the fans was the main X, the results were clear. “After 18 fan failures in two years, we went four and a half months without a rejection,” said Max Limb, a supplier field service representative. “We haven’t completely eliminated the rejections, but we’re close.”
“Our team has become well-versed in the concept of Six Sigma,” said Valerie Feiberti, Supply Management and Procurement Director of the Lean Promotion Team. “We feel very strongly that it provides a way to correct production-related problems and proactively design-in quality.”
Summary
The DMAIC methodology is essentially a series of common sense questions to determine root-cause analysis, identification of X(s), elimination of problems, and maintaining of gains. The DMAIC process asks the following questions:
- Define: What is the Y that is performing poorly?
- Measure: What is Y’s current performance?
- Analyze: What are the X(s)? Are they real?
- Improve: How can X(s) be controlled/eliminated?
- Control: How can X(s) be controlled to sustain gains in Y?
Six Sigma problem solving is the data-driven representation of the conscious or unconscious thinking we use to solve problems in our lives and can be used to solve may needle-in-a-haystack problems that vex businesses.