by Elizabeth Hines | Apr 6, 2015 | Big Data, Blog, Data/Analytics
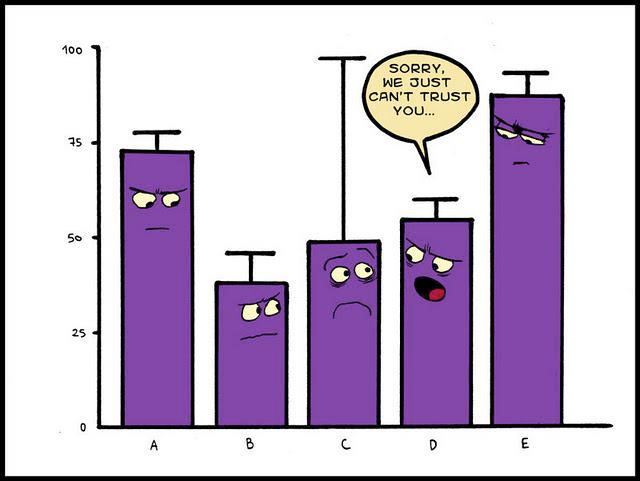
Concurrent with the extraordinary rise of the Internet of Things (IoT), predictive analytics are gaining in popularity. With an increasing number of companies learning to master the precursors to developing predictive models — namely, connecting, monitoring, and analyzing — we can safely assume the art of gleaning business intelligence from foresight will continue to grow rapidly.
Indeed, Gartner analysts put forward that “few technology areas will have greater potential to improve the financial performance and position of a commercial global enterprise than predictive analytics.” And executives seem eager to jump on the bandwagon; in a survey of executives conducted by Accenture a full 88% indicated big data analytics is a top priority for their company. Amid the promises of predictive analytics, however, we also find a number of pitfalls. Some experts caution there are situations when predictive analytics techniques can prove inadequate, if not useless.
Here, we dissect three problems most commonly encountered by companies employing predictive analytics.
Past as a poor predictor of the future
The concept of predictive analytics is predicated on the assumption that future behavior can be more or less determined by examining past behaviors; that is, predictive analytics works well in a stable environment in which the future of the business is likely to resemble its past and present. But Harvard Business School professor Clayton Christensen points out that in the event of a major disruption, the past will do a poor job of foreshadowing future events. As an example, he cites the advent of PCs and commodity servers, arguing computer vendors who specialized in minicomputers in the 1980s couldn’t possibly have predicted their sales impact, since they were innovations and there was no data to analyze.
Interpreting bias
Consider also the bias in favor of a positive result when interpreting data for predictive purposes; it is one of the most common errors in predictive analytics projects. Speaking at the 2014 Predictive Analytics World conference in Boston, John Elder, president of consulting firm Elder Research, Inc., made a good point when he noted that people “‘often look for data to justify our decisions,’ when it should be the other way around.”
Collecting and analyzing unhelpful or superfluous data
Failure to tie data efforts to operational processes can lead to an unnecessary drain of staff resources. Mining big data will do little good if the insights are not directly tied to functional procedures. More companies than we probably realize are wasting precious time and manpower on big data projects that are not adequately understood, producing trivia rather than actionable business intelligence.
To overcome these common pitfalls of predictive analytics, spend some time reviewing the sources of your data and the basic assumptions on which your predictive analytics projects are based. Because the major principle of predictive analytics is that the past behavior can forecast future behavior, keep your ear to the ground for growing industry trends or any other factors that might influence consumer behavior. Plan to revisit the source of your data frequently to determine if the sample set is representative of your future set and should continue to be used. Most importantly, regularly evaluate how your predictive analysis relates and contributes to your company’s overall goals and objectives.
What else do you think organizations can do to ward off the snags of predictive data analysis and use foresight more effectively?
by Elizabeth Hines | Apr 1, 2015 | Big Data, Blog, Data/Analytics, Logistics, Supply Chain
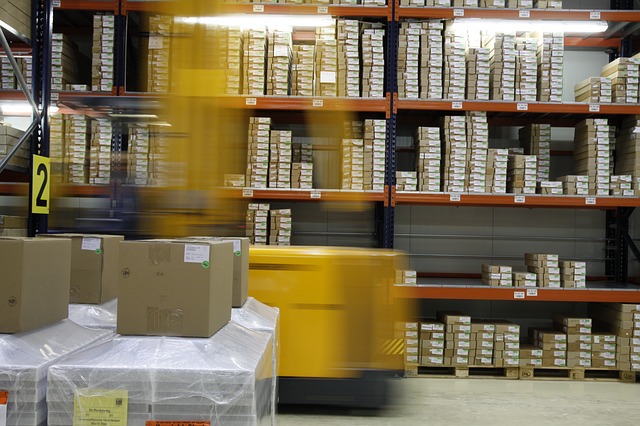
A distribution center struggling with a high number of forklift truck impacts found a way to leverage big data to address a nagging, costly warehouse issue. The company had installed a telematics solution on its forklift trucks, but could not determine the cause of the impacts. The time and location of impacts were known, as well as the identity of the drivers involved, but the company still needed to pull in more data sources for an effective assessment.
Forget for a moment the potential of adopting big data analytics throughout the entire supply chain and consider instead how big data can untangle and integrate seemingly unrelated masses of data to solve small problems in a warehouse or distribution center. That’s exactly what this company did.
By analyzing the link between environmental factors inside the distribution center and the forklift impact records, the culprit was swiftly identified: fast-moving thunderstorms that caused the humidity level to rise so quickly that the dehumidifiers could not keep up, increasing the risk of drivers losing control on the slippery concrete floor. That knowledge helped the company prevent sliding accidents by using a function of the telematics solution to reduce the maximum speed of the trucks when the humidity hit a certain level.
Indeed, distribution centers and warehouses present ideal environments — microcosms — for big data applications. Modern facilities are loaded with sensors and detectors to track every pallet and every piece of material handling equipment in real-time. Managers see the benefits in increased productivity, improved inventory flow, optimized equipment usage, and more. However, for that Eureka moment, organizations should also apply big data analytics across these multiple sources of data to uncover patterns that will drive even more, and perhaps surprising, operational improvements.
Rather than looking at data in isolation, a holistic approach holds significantly more power. Managers typically keep careful track of the performance of lift trucks, batteries, and chargers. But it is not until those entities are reviewed as a single system and matched with data coming off the lift trucks that a new level of revelations can be had.
Look for big data analytics to further raise the IQ of our “smart” warehouses and DCs. Inbound Logistics sums it up this way: “Accessing the right information to make smart decisions in the warehouse is one main reason why the demand for big data has grown so much — and so rapidly — in the distribution sector.”
Do you think distribution center and warehouse managers do enough to leverage big data?
by Elizabeth Hines | Apr 1, 2015 | Big Data, Blog, Data/Analytics, Logistics, Supply Chain
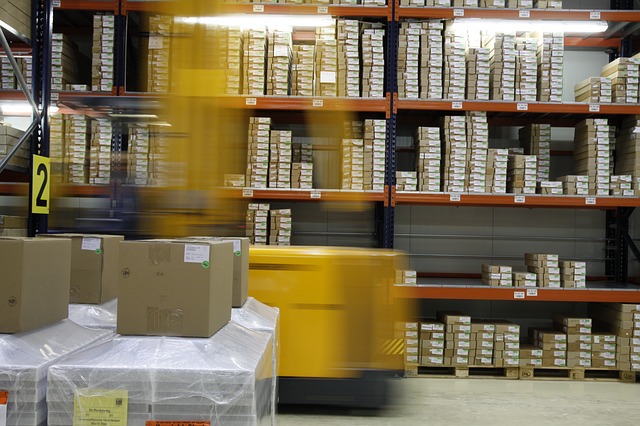
A distribution center struggling with a high number of forklift truck impacts found a way to leverage big data to address a nagging, costly warehouse issue. The company had installed a telematics solution on its forklift trucks, but could not determine the cause of the impacts. The time and location of impacts were known, as well as the identity of the drivers involved, but the company still needed to pull in more data sources for an effective assessment.
Forget for a moment the potential of adopting big data analytics throughout the entire supply chain and consider instead how big data can untangle and integrate seemingly unrelated masses of data to solve small problems in a warehouse or distribution center. That’s exactly what this company did.
By analyzing the link between environmental factors inside the distribution center and the forklift impact records, the culprit was swiftly identified: fast-moving thunderstorms that caused the humidity level to rise so quickly that the dehumidifiers could not keep up, increasing the risk of drivers losing control on the slippery concrete floor. That knowledge helped the company prevent sliding accidents by using a function of the telematics solution to reduce the maximum speed of the trucks when the humidity hit a certain level.
Indeed, distribution centers and warehouses present ideal environments — microcosms — for big data applications. Modern facilities are loaded with sensors and detectors to track every pallet and every piece of material handling equipment in real-time. Managers see the benefits in increased productivity, improved inventory flow, optimized equipment usage, and more. However, for that Eureka moment, organizations should also apply big data analytics across these multiple sources of data to uncover patterns that will drive even more, and perhaps surprising, operational improvements.
Rather than looking at data in isolation, a holistic approach holds significantly more power. Managers typically keep careful track of the performance of lift trucks, batteries, and chargers. But it is not until those entities are reviewed as a single system and matched with data coming off the lift trucks that a new level of revelations can be had.
Look for big data analytics to further raise the IQ of our “smart” warehouses and DCs. Inbound Logistics sums it up this way: “Accessing the right information to make smart decisions in the warehouse is one main reason why the demand for big data has grown so much — and so rapidly — in the distribution sector.”
Do you think distribution center and warehouse managers do enough to leverage big data?
by Elizabeth Hines | Sep 25, 2014 | Blog, Strategy, Supply Chain
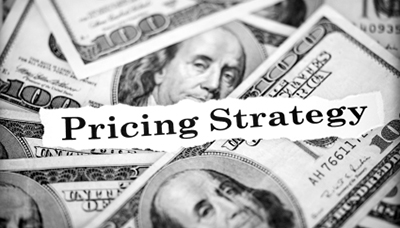
Repeat customers contribute to long-term profitability. To keep customers coming back you need to employ the right pricing strategy. What is the right strategy? Here is what to consider when setting prices:
Focus on value pricing
Value pricing is not the same as discount pricing. Rather, value pricing is pricing a product or a service based on what the customer believes it is worth. Price Intelligently puts it this way: “Customers don’t care how much something cost you to make or your competitors, they care how much value they’re receiving at a particular price.”
Figuring what your target customers believe should be the right price takes a lot of time and research. Because of this, many companies steer clear of value pricing. However, by focusing on value pricing you will be able to increase your long-term profitability, gain customers, and develop a following of loyal customers.
Neil Baron wrote a great article for Fast Company on how a dead squirrel taught him about value pricing. The long and short of it, according to Baron: “Many companies worry about the commoditization of their offerings and their inability to justify premium pricing — but if you figure out how to take care of your customers’ ‘dead squirrels,’ you’re golden.”
Make pricing flexible
Once you have determined your value price, don’t be rigid. Rather, set the price with a modicum of flexibility. Even a little flexibility will go a long way in terms of being able to adjust to customer’s needs and the needs of your company.
Eliminate complexity
Complex pricing not only creates a hosts of challenges, it also undermines the credibility of your company and turns off/away customers. Your pricing should be fair, direct, and easy to understand – both internally and externally.
By keeping these things in mind, you should be able to create a pricing strategy that will attract and retain customers – essential components of long-term profitability.
by Elizabeth Hines | Sep 11, 2014 | Blog, Strategy, Supply Chain
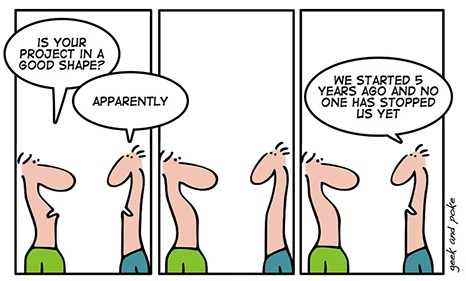
Strategy is not execution
Strategy and execution are fundamentally different. Strategy is about making choices. Execution is about getting down and dirty so that the choices made can produce results. Here’s how you can get from excellent strategy to strong execution.
Strategy is the pursuit of excellence. It is more than white boards, spreadsheets, taglines, slogans, and vanity metrics. Strategy is about making choices on where to play, how to play, and how to maximize value — for your company and for your customers.
Execution is about doing the work needed to produce results within the context of the aforementioned strategy.
Here is how to move from strategy to execution.
Keep it simple.
When it comes to strategy simplicity is key. Develop a few simple but big ideas or themes that will drive your organization. Live or die by these ideas. If you develop too many or they are too complicated, you will lose focus and internal buy-in. When you lose focus and internal buy-in, execution is doomed to failure.
Involve your team.
When you pick your few big ideas (see above), make sure you involve your “front line” team. These are the people who turn action into daily routines. Their insight and knowledge are invaluable.
Be obsessed with your strategy.
If you cannot articulate the “why” of your strategy in everything your organization does, it is probably the wrong strategy. Being obsessed with your strategy means that your strategy can be demonstrated in every action, every day.
Focus on the customer.
If it is not about the customer, it is not strategic. Everything you do needs to link back to the people who are paying you… your customers. If it does not help them, it does not help you and therefore cannot be strategic.
By staying focused on these business execution strategies, you’ll be able to successfully move your supply chains from idea to reality.